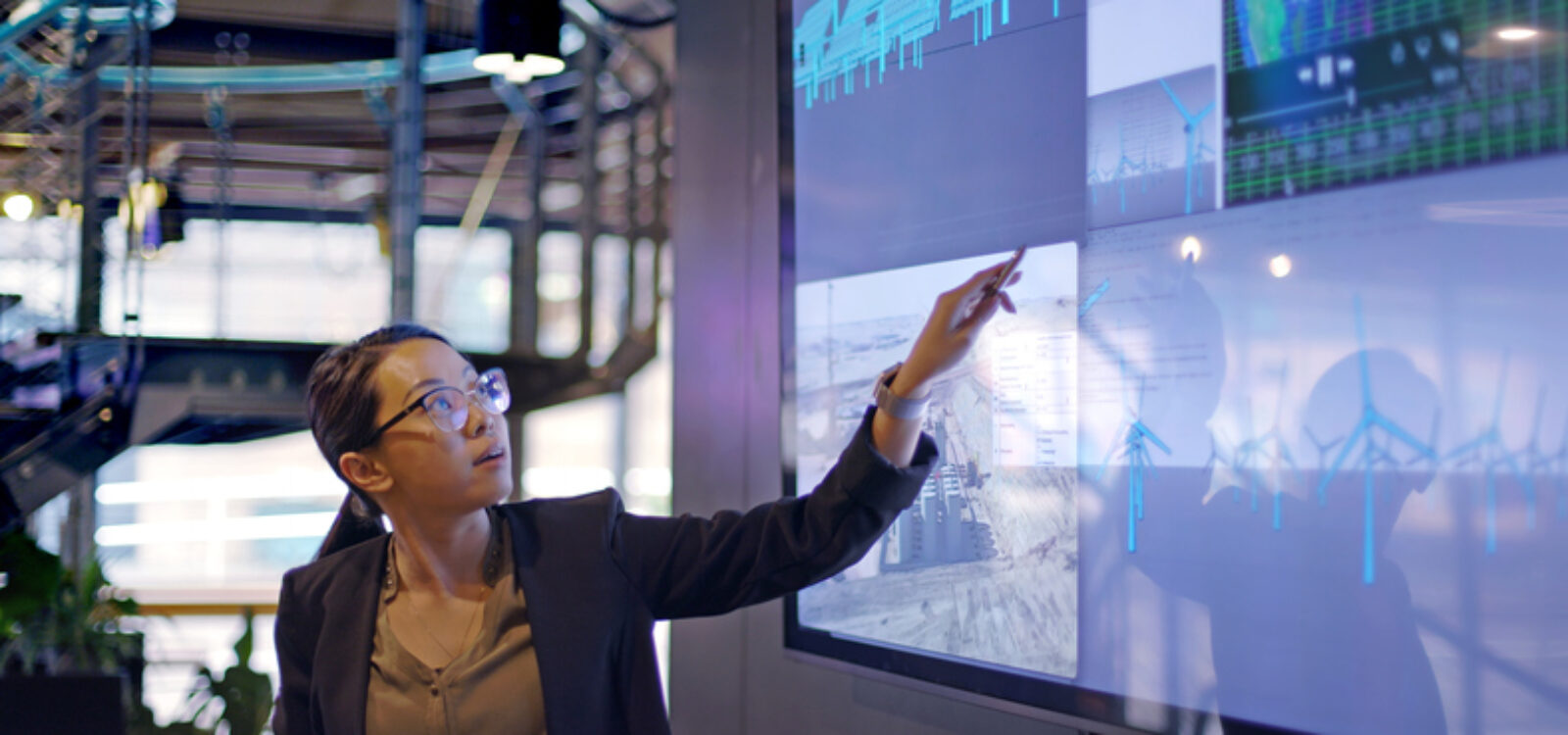
Are correlations real or imagined?
Actuaries Benjamin Avanzi, Greg Taylor and Bernard Wong challenge preconceptions regarding the high level of dependence between general insurance claims from different classes. Using real life data, they show how more detailed analyses can often reveal systemic effects, such as weather events or seasonality, which can account for much of the observed correlation, leaving much lower levels of residual correlation.
Background
In August 2014, we outlined the main objectives of a research project carried out by us and our team of researchers, in collaboration with Allianz, Insurance Australia Group, and Suncorp1. The focus of the project is on claim dependencies, and one of the great outcomes of our collaboration with the industry is the setting up an extensive data base of real insurance claims at the single transaction level. This will be referred to as the “AUSI” data set (Allianz, UNSW, Suncorp and IAG).
In actuarial practice, correlations are widely used to represent dependencies. Furthermore, there seems to be a great deal of preconceptions on how dependent insurance claims should be. Often, the presence of dependence is taken as a given. Dependency is rarely discussed or challenged, perhaps because of the lack of an extensive public dataset and research on the actual dependencies shown in this dataset.
Australian actuaries working in the general insurance field would be familiar with the correlation matrices developed in Bateup and Reed (2001)2 or Collings and White (2001)3 for the purpose of assessing risk margins for insurers. The correlations contained there are based largely on the judgement of a small number of actuaries, with limited under-pinning in data (in one case none). A number of the correlations are of considerable magnitude, with little factual evidence to support them, and are possibly overstated, resulting in deliberately conservative risk margins.
Other references relevant to the construction of risk margins generally are O’Dowd et al. (2005)4 and the Risk Margins Task Force (2008)5.
Our recent work
In a recent paper (Avanzi, Taylor and Wong, 20156), we develop a simple theoretical framework that enables us to explain rigorously how and why correlations can be illusory (and what we mean by that). In the present short article, we report on some of the pertinent results we obtained when analysing the AUSI data set. These have material implications on the estimation of diversification benefits (and hence adequate capitalisation), and the modelling of dependencies in general.
Correlations in the AUSI data set
How much correlation does the AUSI dataset actually display? We extracted from the AUSI data set six claim triangles. These span 40 quarters (2004 to 2013 inclusive) and originate from two insurers (“Insurers A and B). The Lines of Business (“LoB”) we considered are Home, Private Motor, CTP, and Public liability (note that Home and Private Motor data were not available for Insurer B at the time of the analysis).
Simple chain ladder modelling was carried out, and cross-LoB correlation calculated for each within-insurer pair of LoBs. The results are shown in Table 1. It is remarkable that most correlations are very weak, with the notable exception of Home and Motor for Insurer A. Can we then conclude that Home and Motor are highly dependent?
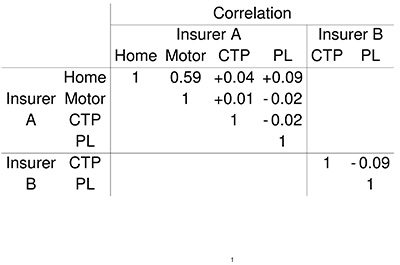
Illusory correlations and modelling
Jumping to this conclusion is very tempting. However, this would ignore one of the fundamental rules of modelling, which is to search for influential covariates that are missing from the model, and then include them. Such a routine has potential to wipe out some, if not all, of the observed correlation.
Time series should be de-trended before estimation of correlation between them. Otherwise, there is a risk of estimating high (or low) correlation simply because the series trend in the same direction or opposite directions.
For many applications, correlation between two variates relates to the stochastic noise contained in them. These are the components of the variates that are, by their nature, not capable of being modelled. In such cases, one should model all systematic (non-stochastic) effects that are identifiable in the observations, remove these effects, and correlate the remainders of the observations.
Case study: Home and Motor
The significant correlation displayed between Home and Motor for Insurer A provides an ideal case study.
The briefest examination of the data indicates substantial differences between accident periods in the total volume of claims. Figure 1 charts, for each of development quarters one to three, the time series across accident quarters, of the cumulative proportion paid of Home loss payments made in those quarters (relative to the total over the first eight development quarters). This chart also displays, by accident quarter, the total of Home claim payments made in the first eight development quarters . These plots are characterized by occasional very marked peaks, which can be confidently attributed to major weather events. Some peaks affect both LoBs (e.g. in accident quarters 16, 25 and 32). These will have generated large positive residuals in both LoBs, increasing measured correlation. They will also have created a tendency in both LoBs toward negative residuals in most of the other accident quarters, further increasing correlation.
Figure 1: Volume of claims paid by accident quarter and effect on paid loss development
Figure 1 also suggests seasonal influences. There is a hint of slower payment of claims in every fourth accident quarter, specifically quarters numbered four, eight, etc. It is also noticeable that these accident quarters are marked by generally higher claim payments. This effect is somewhat confounded by the occurrence of major events, whose accident quarters are marked by especially high claim payments and slow rates of claim payment. It is reasonable to suppose that the claims of any particular accident quarter are likely to be paid more slowly (or rapidly) according as the volume of claims impacting the accident quarter is high (or low). One might therefore infer a systematic effect according to which each fourth accident quarter (actually the fourth accident quarter of the calendar year, late spring and early summer) coincides with increased claims activity, probably weather related, and retarded processing of claims. In fact, a closer examination of the data indicates that a similar effect occurs in the first quarter of the calendar year (most of summer and early autumn).
When we included weather events in the modelling, by deleting accident quarters subject to extraordinarily heavy paid losses, correlation decreased from 0.59 to 0.11. Further recognition of the seasonal effects hypothesised above led to a residual correlation of -0.01. These results are compelling, and more dramatic than we had initially expected.
We should note that in Avanzi, Taylor and Wong (2015)7 we performed a similar analysis on some Schedule P data from the United States, with similar results.
A word of caution
There is a fundamental difference in the required treatments of past and future data. While past data may be sufficient for the identification of specific effects contained within it—that is, sufficient for any apparent cross-LoB correlations to be modelled away—the same is not necessarily true of future (i.e. unobserved) data. Even if past observations are free of correlation, this may provide only a benchmark in relation to future data. Unobserved future errors (e.g. in superimposed inflation, or claim frequency induced by major events) may well be correlated as between LoBs, in which case it may be appropriate to allow for non-zero correlations.
Of the factors found in this article to generate apparent correlation, the first (seasonal effects), once modelled in past data, requires no further action. Any sympathetic trends in the cash flows of separate LoBs will be automatically contained in the model forecasts without resort to the use of correlation.
The treatment of the second factor (major events) would be different. The effects of these have been removed from the models discussed above, and so would be excluded from those models’ forecasts. They would typically be restored by means of CAT model forecasts, applied simultaneously across LoBs, and so restoring dependency between them. Note, however, that the degree of dependency in forecasts may differ from that observed in the past if the modelled data set is atypical with respect to major events.
Conclusions
Two major conclusions are drawn:
- In any attempt to measure cross-LoB correlations, the data needs to be modelled very carefully. The exercise will not always be well served by rough modelling, such as the use of simple chain ladders, and may indeed result in the prescription of excessive risk margins and/or capital margins.
- Such empirical evidence as examined here reveals cross-LoB correlations that vary only in the range zero to very modest. There is little evidence in favour of the high correlation that is assumed in some jurisdictions. The evidence suggests that these assumptions derived from either poor modelling or a misconception of the cross-LoB dependencies relevant to the purpose to which they are applied.
There still is a lot to be done. We merely illustrate here that correlation levels are model-dependent. They mostly originate from unaccounted effects. The recognition of those, and their appropriate modelling, represent major challenges. Furthermore, in some cases correlations may not be an appropriate representation of dependencies (especially in the tails). We will be back.
Register now for the upcoming Insights session with Greg, Bernard and Benjamin at the Actuaries Institute in Sydney, or via webinar, on 23 October 2015.
1Avanzi, B., Taylor, G., Wong, B. (2014) Research into Claim Dependencies – an Industry and Academic Collaboration. The Actuaries Magazine, August 2014, pp 9-11. Available at http://www.actuaries.digital/2014/08/15/research-into-claim-dependencies-an-industry-and-academic-collaboration/.
2Bateup, R., Reed, I., 2001. Research and data analysis relevant to the development of standards and guidelines on liability valuation for general insurance. The Institute of Actuaries of Australia and Tilinghast – Towers Perrin.
3Collings, S., White, G., 2001. Apra risk margin analysis. In: Institute of Actuaries of Australia (Ed.), XIIIth General Insurance Seminar.
4O’Dowd, C., Smith, A., Hardy, P., 2005. A framework for estimating uncertainty in insurance claims cost. In: Institute of Actuaries of Australia (Ed.), XVth General Insurance Seminar.
5Risk Margins Task Force, 2008. A framework for assessing risk margins. In: Institute of Actuaries of Australia (Ed.), XVIth General Insurance Seminar.
6Avanzi, B., Taylor, G., Wong, B. (2015) Correlations between insurance lines of business: An illusion or a real phenomenon? Some methodological considerations. UNSW Business School Research Paper No. 2015ACTL11. Available at http://papers.ssrn.com/abstract=2597405.
7Note that the relevant axis for this series of data, in $, would normally appear on the right hand side of the plots. We had to redact it for confidentiality reasons.
CPD: Actuaries Institute Members can claim two CPD points for every hour of reading articles on Actuaries Digital.