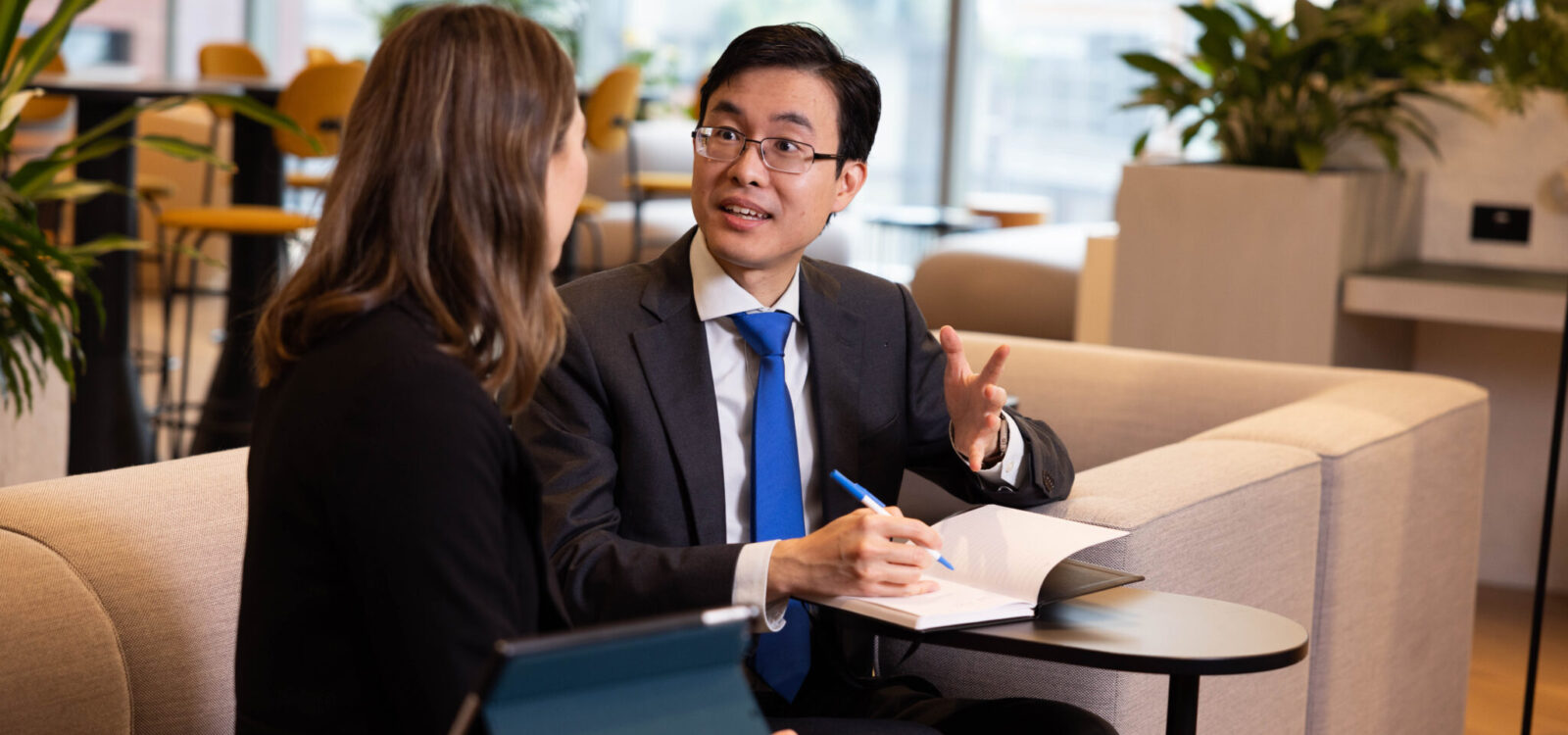
What makes a good data scientist? – Actuaries Podcast
Effective data scientists need to understand the workings of IT teams and identify the limits of truth in models, says Co-Founder and Chief Scientist at Ambiata.
Tiberio was interviewed by Anthony Tockar on the Actuaries Institute’s podcast last month. This is the second episode from that conversation (the first explored the ethics of machine learning).
Listen to “What Makes a Good Data Scientist?” on Spreaker.
If an actuary is working as a data scientist for a large organisation with millions of customers and complex internal IT legacy systems, being able to engage well with internal IT and data teams is critical. That means, as a first step, knowing their lingo.
“You don’t have to be a database engineer [or] a Hadoop expert or anything to work with those technologies and work with those teams, but you need to be speaking the same language essentially,” said Anthony.
Data science combines both statistics and computer science. Actuaries are skilled in the former through their modelling and forecasting work, for the latter, they must upskill. As an actuary who transitioned into data science, Anthony said he spent most of his learning time on computer science. He highlights the importance of having a good handle on the underlying flow of data that informs the modelling.
“When you want to deploy things at scale, it’s really important to have familiarity with data pipelines and understanding of databases [and] of the new thinking around ‘how do you manage data?’ says Tiberio Caetano.
From academia to ‘the wild’
After studying engineering and physics, then taking a PhD in computer science, Tiberio became fascinated with computer vision and machine learning.
From Brazil, then Canada, he came to Canberra, Australia to work alongside hundreds of PhDs from all over the world at NICTA – Australia’s Information and Communications Technology Research Centre (which merged with CSIRO in 2016).
“Forget crazy robots taking over the world, forget dystopian or utopian futures – machine learning is about providing a machine a description of your goal and let the machine work out by itself the steps required to achieve that goal.” – Tiberio Caetano
Wanting to see what an academic could achieve “in the wild”, Tiberio co-founded Ambiata, a company that applies machine learning and predictive analytics to media, government, insurance, banking, telecommunications and retail businesses.
“That was a very deep sort of incursion into reality that not many academics get to indulge in,” said Tiberio, adding how hard it was to transform ideas into practical outcomes, but also how gratifying it was when it was possible.
Knowing your limits and quantifying uncertainty
When asked ‘what makes a good data practitioner?’ Tiberio says it’s knowing the limits of your knowledge and how to accurately estimate uncertainty in models:
“Most problems I see resulting from data science action come from mis-assessment of your knowledge about something: how little you know about how much you know,” says Tiberio. “You need to be critical about everything, but especially about your own ideas.”
When building pricing models, Tiberio suggested actuaries should work to understand how error bars, significance tests and every other technicality translates in layman’s terms.
“They are models, they are not the truth. The truth is much more complex, and you need to have certain ways to assess the extent to which your model is different from the truth.
“You have ‘model uncertainty’ which is basically the bias that you are introducing when you create a model because you don’t know the truth … and you have ‘statistical uncertainty’ which is the fact that you don’t have all the data in the world, you just have a finite sample.
“Learn more about how to quantify uncertainty; how to question your models and be more accurate about certainty in your models,” he said.
Skills
Building your skill-set out
Skills data scientists need cover programming, algorithms, machine learning, statistics, visualisation, reporting and data management. Some people are able to cover a range of topics, but in other cases the best option is to build a good team that covers the skills.
An entrepreneurial mindset that is constantly learning, passionate and innovative is also important for data scientists; whatever they build may be obsolete in a couple of years as better solutions are developed.
Communication is equally important. As Anthony said in an earlier presentation, data scientists infer and explore the data, and explain what’s happening to the business, often through visualisation. They often face challenges in explaining experimentation to non-scientists and knowing their audience. Daniel Marlay, Director at EY, provides some tips in this presentation on how to give either an outcome focus to senior management or use a narrative style with lots of detail when communicating to more technical audiences.
Tiberio will give the Closing Keynote Address “Ethics behind AI and Technology” at the 2018
General Insurance Seminar (12-13 November).
View the program and register to attend – one and two day tickets are available.
Useful links and resources
Data Analytics Newsletters:
- August 2018 – Analytics in the new actuarial education pathways + News and Tutorials
- September 2018 – Ethical issues in data
- October 2018 – Careers in Data
Presentations from the 2018 Data Analytics Seminar on Wednesday 31 October will be available following the Seminar on the Program Snapshot page.
Insights – What is Data Science? Tuesday 12 September 2017, Sydney. Presented by Anthony Tockar
Presentations from the inaugural Data Seminar (2015) – members only.
Finding the Data Analytics Unicorn Event Report on 2015 Data Seminar by Amanda Aitken
CareerView Podcast – Managing a Data Analytics Team, with Hugh Millerand Aaron Cutter
Actuaries Podcast – the ethics of machine learning, with Tiberio Caetano and Anthony Tockar
How actuaries can get started in analytics, Normal Deviance column by Hugh Miller
CPD: Actuaries Institute Members can claim two CPD points for every podcast listened to.