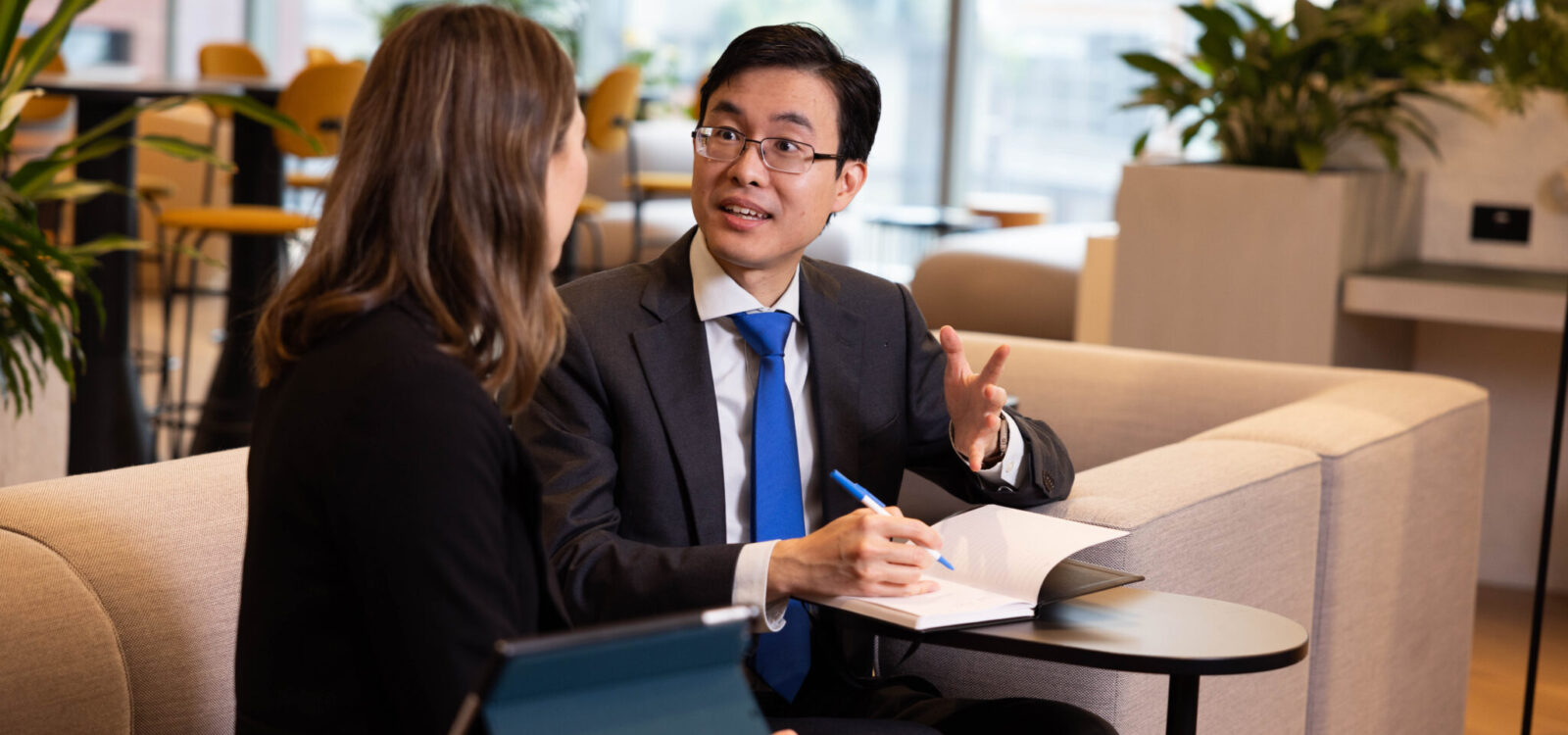
Risk margins with false correlation matrices
Many of the general insurance risk margins constructed in practice are based on flawed correlation matrices. Here, Greg Taylor examines two industry approaches to the construction of large correlation matrices for risk margin purposes.
The following article is based on an Actuaries Institute Insights presentation, which can be found here. This, in turn was based on a formal research paper (Taylor, 2018), a pre-print of which can be found here.
Many of the general insurance risk margins constructed in practice are based on flawed correlation matrices. In my paper, two industry approaches to the construction of large correlation matrices for risk margin purposes have been examined, one of them endorsed by European Insurance and Occupational Pensions Authority (EIOPA).
The problem
Construction of a risk margin that spans a number of business segments usually requires that any stochastic dependencies (or lack thereof) between those segments be taken into account. These dependencies are typically accounted for by means of a correlation matrix. In this article, this usage of correlation matrices is accepted as a given, and its appropriateness set aside as a subject that has been discussed elsewhere.
This becomes a substantial issue when the segments are layered and/or numerous. For example, a global insurer might layer its business hierarchically by:
- global region;
- nation;
- state (within nation);
- source of business;
- line of business (LoBs).
Such a structure generates many business segments, and the associated correlation matrix can become very large and cumbersome. If the number of segments is $$\mathcal{n}$$ then the number of separate correlation coefficients to be estimated is $$\frac{1}{2} n(n – 1)$$
Some approaches to the problem found in practice
I have seen a couple of approaches used in practice.
Approach 1
Avoid the construction of the full correlation matrix. Instead, formulate a number of smaller correlation matrices: one for just global regions, then one for the nations within each global region, one for the states within each nation, and so on.
Use these to aggregate standard deviations (SDs) of technical reserves upward. Starting at the lowest level of the hierarchy, aggregate the SDs of the LoBs into an SD for a source of business, taking account of the correlation matrix over the LoBs.
Then aggregate SDs for sources of business into SDs for states. And so on, until all regions have been aggregated into a global SD. Call this the hierarchical algorithm.
This approach is indeed the one generally accepted to be recommended by EIOPA for Solvency II (though the actual text may not be so unambiguous).
Approach 2
Again, this consists of formulating a collection of smaller matrices, in this case a region-nation-state matrix, a source matrix, and an LoB matrix, which are used to generate the full correlation matrix.
As an example, the correlation between (region-nation-state $$i_1$$, source $$j_1$$, LoB $$k_1$$) and (region-nation-state $$i_2$$, source $$j_2$$, LoB $$k_2$$) is calculated from the smaller matrices as
$$\begin{align*}\rho^{region-nation-state}_{i_1 i_2} \rho^{source}_{j_1 j_2} \rho^{LoB}_{k_1 k_2}\end{align*}$$
where e.g. $$\rho^{LoB}_{k_1 k_2}$$ denotes the correlation between LoBs $$k_1$$ and $$k_2$$, etc.
In technical terms, the full correlation matrix is the tensor product of the smaller matrices.
A requirement of any approach
There is a basic requirement that any approach to aggregation of business segments must satisfy. It is the following:
$$\begin{align*}\text{Var}[Y] = \sum_{i=1}^n \text{Var}[Y_i] + \sum_{\substack{i,j=1 \\ i \neq j}}^n \text{Corr}[Y_i,Y_j] \sqrt{\text{Var}[Y_i]\text{Var}[Y_j]}\end{align*} \\$$
where there are $$n$$ indivisible business segments (those at the lowest level of the hierarchy), $$Y_i$$is the technical reserve for business segment $$n$$, and $$Y = \sum_{i=1}^n Y_i$$, the total reserve over all segments. Call this the composability requirement.
If an approach does not meet this requirement, then it is difficult to regard it as generating a valid variance of total technical reserve.
Validity of the suggested approaches
It is necessary to check whether Approaches 1 and 2 satisfy the composability requirement.
Approach 1
In this case, it is possible to establish the following interesting precise mathematical result. It is stated as a “theorem”, but in fact the statement is slightly popularised for readability. See the full paper for a tighter statement.
Theorem
Suppose that the insurer’s business segments form a tree of at least order 2 (i.e. the node of the tree has at least a child and a grand-child. Now consider a node $$\mathcal{N}$$ somewhere in the tree, and construct its variance by means of the hierarchical algorithm
Suppose that:
- Every non-terminal node in the hierarchy has at least two descendants; and
- None of the “intermediate” correlation matrices at $$\mathcal{N}$$ or any of its descendant nodes consists entirely of unit entries.
Then a necessary and sufficient condition that the technical reserve variance aggregated to node $$\mathcal{N}$$ satisfy the composability requirement is that there are no correlations between segments at any descendant nodes of $$\mathcal{N}$$ other than possibly the leaves of the tree.</p
Corollary. Take node $$\mathcal{N}$$ to be the root node (total portfolio). Then the necessary and sufficient condition is that there are no correlations between segments at any nodes of the entire hierarchy other than possibly the leaves.
According to these results, if the composability requirement is to be satisfied, the only correlation matrices admitted at nodes of the tree other than the leaves are those involving no correlations at all or perfect correlations between segments. Trivial dependency frameworks of this sort are not useful, and so proposed Approach 1 does not produce a valid portfolio variance in any realistic situation.
Approach 2
The tensor product of a collection of correlation matrices is indeed itself a correlation matrix. However, the problem that arises here is one of interpretability of the factor matrices.
For example, the factor $$\rho^{Source}_{j_1 j_2}$$ occurred in the above illustration of a tensor product. This purports to be the correlation between technical reserves for sources of business $$j_1$$ and $$j_2$$, say direct and intermediated. But does this make sense? Each of these two sources consists of an aggregate of LoBs, and so the correlation between the two sources will, in general, not be a constant but will vary with changes in LoB mix.
The question then to be considered is whether there exists some model which yields a sensible interpretation of the tensor product of correlation matrices. The paper explores this issue a little. It demonstrates that the most obvious model one might conjecture does not provide a sensible interpretation.
No firm conclusion is reached. No model is found, but nor is a proof given that no model exists. My guess, for what it is worth, is that either no model exists or, if it does, it is so complex or contrived as to be of little practical value.
Conclusion
Two industry approaches to risk margin purposes have been reviewed. The approach endorsed by EIOPA is flawed in that it fails to meet a basic requirement that is, to my mind, essential. The other lies in a limbo state, where its validity is unclear, but its falsity has not been proven. My own guess is that either it too is flawed, or that any version that can be said to have mathematical integrity is not of much practical use.
It might be pointed out that an earlier paper (Avanzi, Taylor & Wong, 2018), discussed in an Insights session, suggested an alternative approach to the same problem, namely the common shock approach. This generates a coherent model.
Acknowledgment
This research was supported by under the Australian Research Council’s Linkage Projects funding scheme (project number LP130100723).
References
Avanzi B, Taylor G & Wong B (2018). Common shock models for claim arrays. Astin Bulletin. In press. Also appears at https://papers.ssrn.com/sol3/papers.cfm?abstract_id=2881058.
Taylor G (2018). Observations on industry practice in the construction of large correlation structures for risk and capital margins. European Actuarial Journal (in press). Appears on-line at http://link.springer.com/article/10.1007/s13385-018-0173-7. Also appears at http://ssrn.com/abstract=3113756.
CPD: Actuaries Institute Members can claim two CPD points for every hour of reading articles on Actuaries Digital.