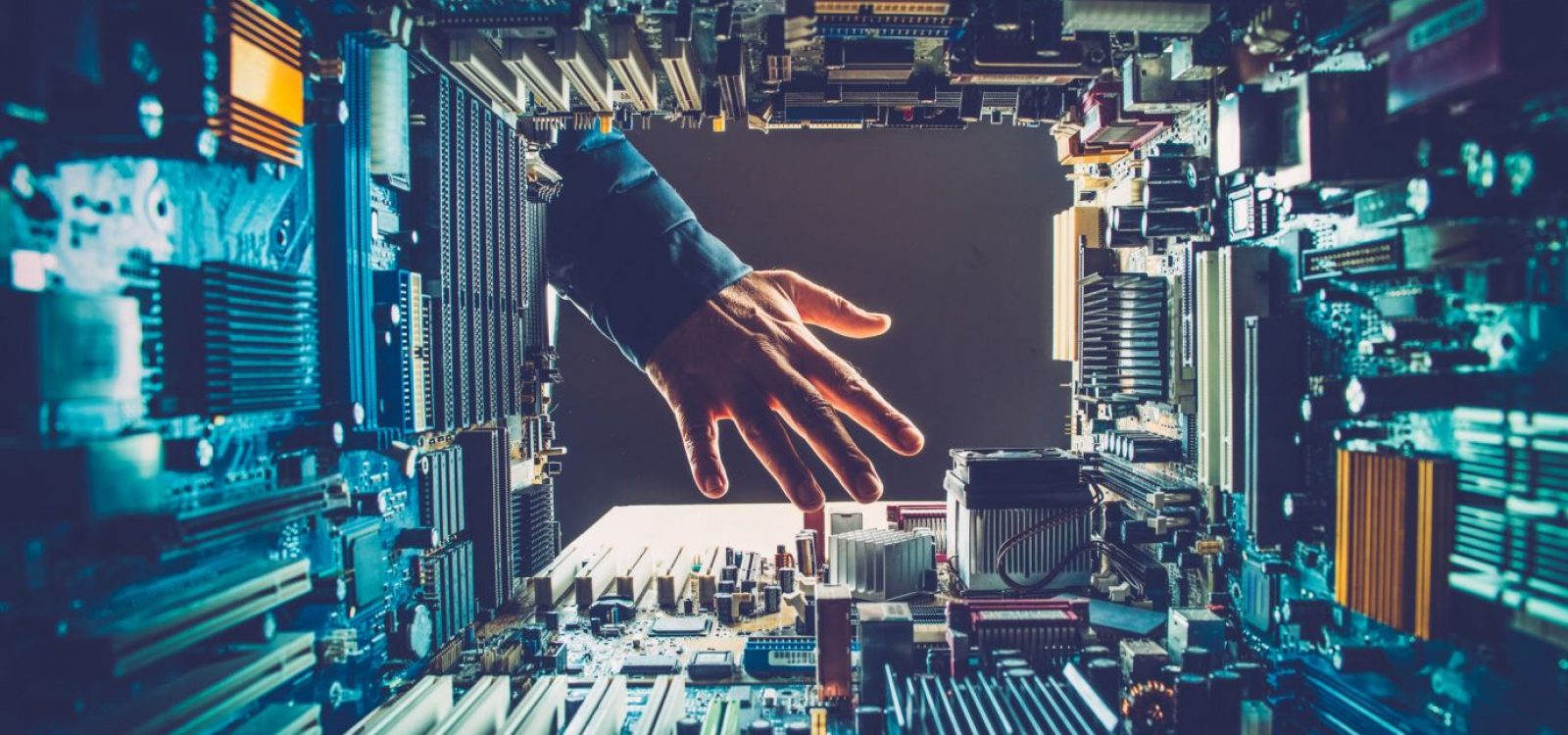
When the algorithm fails to make the grade
In this edition of Normal Deviance, the story of the UK algorithm to assign high school grades following exam cancellations, teaches an important lesson for everyone building models where questions of individual fairness arise.
There have been many consequences of the pandemic. While health and employment concerns are rightly prominent, education is another domain that has seen significant disruption. One recent story intersecting with modelling and analytics is the case of school grade assignment in the UK. With final year exams cancelled due to the pandemic, the Office of Qualifications and Examinations Regulation (Ofqual) was presented with the challenge of assigning student grades, including the A-level grades that determine eligibility for university entrance.
Part of the challenge is that centre-assessed grades (grades issued by schools based on internal assessment) are always optimistic overall compared to actual exam grades, so the process required choosing the best way to move grades closer to historical patterns. An algorithm was created to produce predicted grades across the whole student cohort.
However, when results were posted out there was student outrage at the perceived unfairness of people who received a lower grade than they expected. Pressure led to all governments across the UK backflipping and announcing that centre-assessed grades would be recognized instead of the algorithmic grades. While a win for many students who felt they deserved higher grades, it does raise significant further questions and represents a poke in the eye for those who stood by the robustness of the algorithmic grades.
In many ways the Ofqual algorithm for adjusting grades ticked all the right boxes:
- The process was thorough and transparent, with a detailed report released explaining the methodology, alternatives considered and a range of fairness measures to ensure particular subgroups were not discriminated against.
- The process used available data well, incorporating a combination of teacher-assessed rankings, historical school performance and cohort-specific GCSE (roughly equivalent to our school certificate) performance to produce grade distributions. Such approaches are also used elsewhere. For example, in NSW HSC school assessment grades are moderated down using school rankings so they reflect a cohort’s exam performance.
- The process gave some benefit of the doubt to students, allowing for some degree grade inflation. For courses and school cohorts where there were only a small number of students, more weight was given to centre-assessed grades.
However, with the benefit of hindsight, it was clear that effort was not enough. The main factors contributing to the government backdown:
- The stakes are very high. For many students, the difference between centre-assessed grades and modelled grades is the different between their preferred university degree and an inferior option (or no university admission at all!). Students have a strong incentive to push back on the model.
- Accuracy is good, but it was not great. While the report was careful to describe expected levels of accuracy (and choose methods that delivered relatively high accuracy), the reality is that a very large fraction of students got the ‘wrong’ grade, even if the overall distribution was fair. Variability across exams is substantial, and a very high level of accuracy would be required to neuter criticism and disappointment.
- There were still some material fairness issues. Smaller courses are disproportionately taken by students at independent schools, and under the model these grades were less likely to be scaled back. Thus students attending independent schools were more likely to benefit from leniency provisions.
- The model unilaterally assigned fail grades to students. The modelling included moving a substantial number of people from solid pass grades into the “U” grade (a strong fail grade, literally ‘ungraded’). There’s a natural ethical question whether it is fair to fail students who were not expected to fail according to their teachers, based on school rates of failure in prior years.
- Perhaps most importantly, the approach failed to provide a sense of equality of opportunity. If you went to a school that rarely saw top grades historically, and your school cohort’s GCSE results were similarly unremarkable, there was virtually no way that you could achieve a top grade in the model. This does not sit well with students; the aspiration is that any student should be able to work hard and blitz their exams. Instead, students felt that they were effectively being locked into disadvantage, if they had attended a school with historically lower performance.
Unsurprisingly, the final solution (adopting the centre-assessed grades) will create its own problems. Teacher ‘optimism bias’ is unlikely to be uniform across schools, so students with more realistic teacher grading will be relatively disadvantaged. Teacher grades may be subject to higher levels of gender or ethnic bias. The supply of university will not grow with the increased demand implied by higher grades; in some cases, this may be handled through deferrals which may have knock-on effects for availability for 2021 school finishers. And overall confidence in Ofqual has taken a substantial hit.
I think there are some important lessons here for data analytics more generally. First, models cannot achieve the impossible; in this case, it is impossible to know which students would have achieved a higher or lower mark. In a high-stakes situation, such limitations can break the implementation of a model. Second, it raises the point that something that appears ‘fair’ in aggregate can look very unfair at the individual level.
In situations where individual-level predictions have a significant impact, we should spend time understanding how results will look at that granular level, and who the potential ‘losers’ of a model are. Finally, an algorithm will often become an easy target. As we’ve also seen in COMPASS and robodebt coverage, a faceless decision-making tool carries a high burden of proof to establish its credibility; this requirement applies from initial model design through to results and communication. Appropriate use of modelling is something we will need to continue to strive for in our work.
#InTheNews – “England exams row timeline: was Ofqual warned of algorithm bias?” from @guardian https://t.co/MjCKgyYc9V#NAPCE #pastoralcare #schools #education #teachers #exams #childwelfare #studentwelfare #covid19 #gcses #alevels pic.twitter.com/ruL5QUaBrs
— NAPCE (@NAPCE1) August 21, 2020
UK ditches exam results generated by biased algorithm after student protests https://t.co/ZQtWT1iqJe pic.twitter.com/G6RAldar59
— The Verge (@verge) August 17, 2020
CPD: Actuaries Institute Members can claim two CPD points for every hour of reading articles on Actuaries Digital.