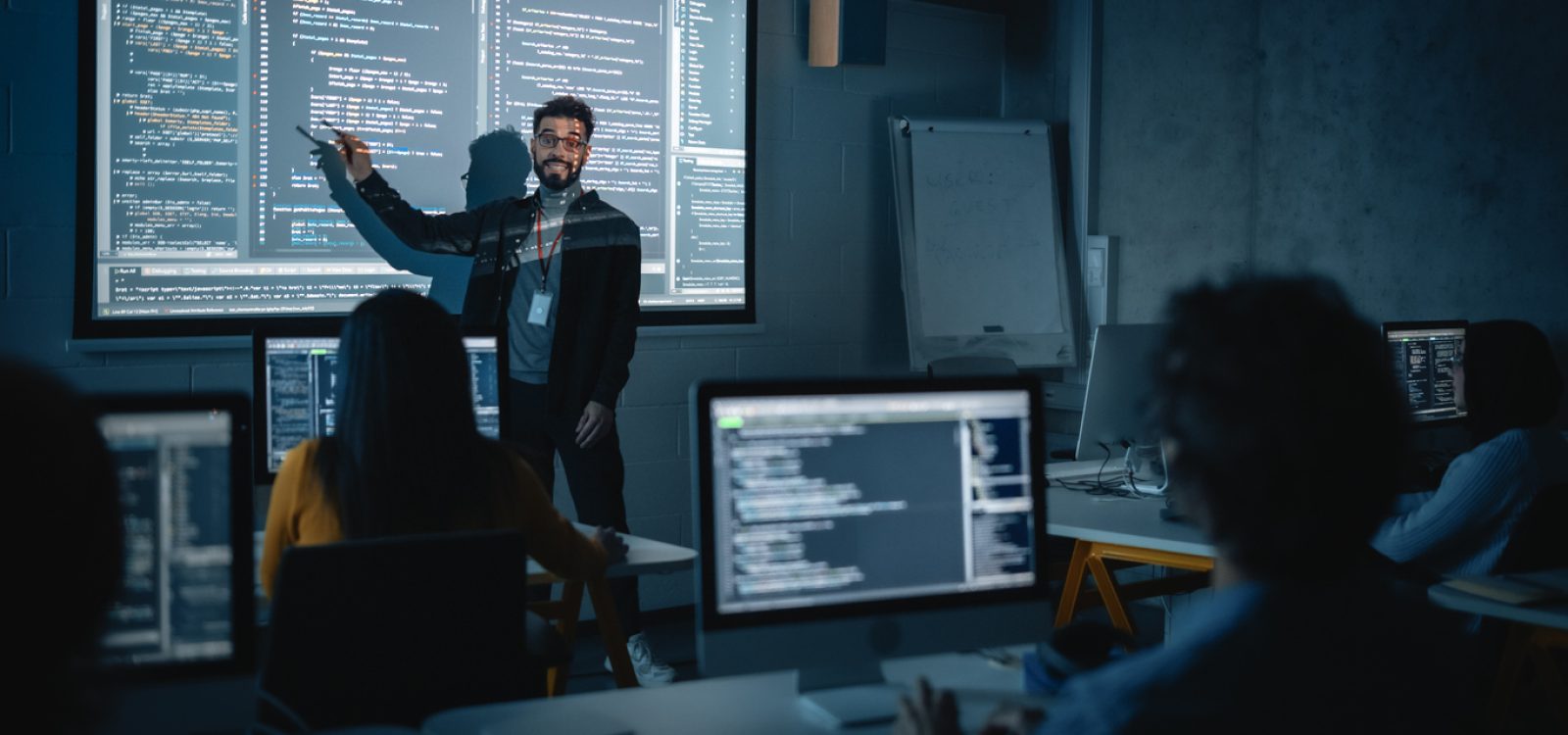
Beware the algorithmic feedback loop
Whenever an algorithmic system is implemented, it changes the underlying experience. Here be dragons.
Algorithms are great. They are the reason why people have engaging, personalised social media feeds, low-risk loan applications can be processed in minutes, and why my YouTube homepage can display an eclectic mix of Eurovision videos, cricket highlights and UK panel show clips.
Virtually all model-based algorithms need to be refined and updated. Actuaries are very familiar with the need to update a model for experience over time. However, this is much more complex when the algorithm’s model is fit using historical data as, after implementation, the algorithm will affect how that data looks in the future. These feedback loops need to be managed carefully, otherwise the next model update jeopardises reinforcing the model judgements, rather than genuinely incorporating new experience.
A case study in the recent book by Jack Maxwell and Joe Tomlinson highlights one of these feedback loops that was built into the UK immigration system in the middle of the last decade. An algorithm assigned risk to each person’s visa application on a three-colour scale system – green, amber and red. The model was built using information on historical visa breaches and included features such as the country of origin.
However, the model was updated over time using both visa breaches and instances where negative immigration decisions were included as breaches. Groups of people flagged as higher risk by the model (based on nationality, for example) were more likely to be rejected by the reviewing officer, and then this rejection reinforces the effect. This outcome feeds into the update and potentially further increases the risk assigned to particular groups and countries. The setup risks a negative spiral of increasingly negative ratings of applicant cohorts over time.
The inclusion of nationality, in and of itself, was problematic, but the feedback loop accentuates the issue. Potential legal challenges, based on accusations of the tool being racist, led to the algorithm being dropped in 2020.
Once alert to the issue of algorithmic feedback loops, the issue is visible in many places. Internet recommendation algorithms, such as those used by YouTube or social media companies, are commonly cited. These can lock users into ‘rabbit holes’ of interest, serving people with a narrow slice of the internet, which can then compound.
The Wall Street Journal undertook an experiment to understand how attention can drive the TikTok recommendation algorithm. The divergences were striking, in one case zeroing in on serving up highly depressive content. By starting with multiple fresh accounts and randomly choosing videos to watch, the recommendations would start to skew towards those previously watched videos.
These feedback loops are particularly important to identify when being applied to triage or decision tools in the public or private sectors. Justice-reoffending risk models that incorporate race (or proxies for it) may be more accurate but also risk reinforcing historical biases such as the over-policing of minority groups.
Insurance pricing models that penalise certain locations for high risk can sometimes reinforce disadvantage, depending on the nature of that historical experience – for example, racial redlining affecting insurance in the USA. Credit models that assess a person’s suitability for a loan can have unfortunate feedback loops if an initial rejection becomes a data point that feeds later decisions.
Even when the impacts are not necessarily bad, the feedback loop can undermine the performance of a model. Any triaging or prioritisation process is subject to this. For example, workers’ compensation triage models that assign different treatment pathways to injured claimants. This occurs by introducing the cohort differences that undermine the ability to refresh the model – the fact that people get different treatments shifts people’s outcomes. For an effective triage model, it may appear that people in the high-risk group for claim duration are no longer as high risk since the triaging has improved their outcomes.
What can we do about the issue?
The most important step is to be aware of whether this issue affects your data. If so, appropriate steps may be obvious. For recommendation algorithms, enough variety is needed in the set of recommendations so that people can ‘escape’ particular rabbit holes they have been sucked into.
For triage and risk models, setting aside a sample as a type of control group (ideally randomised) can help estimate the degree of impact and enable proper adjustment. In cases where the risk and impact of poor predictions is high, the use of algorithms may need to be curtailed.
Algorithms are both useful and inevitable. But because they change our world, we cannot assume they are always neutral.
CPD: Actuaries Institute Members can claim two CPD points for every hour of reading articles on Actuaries Digital.