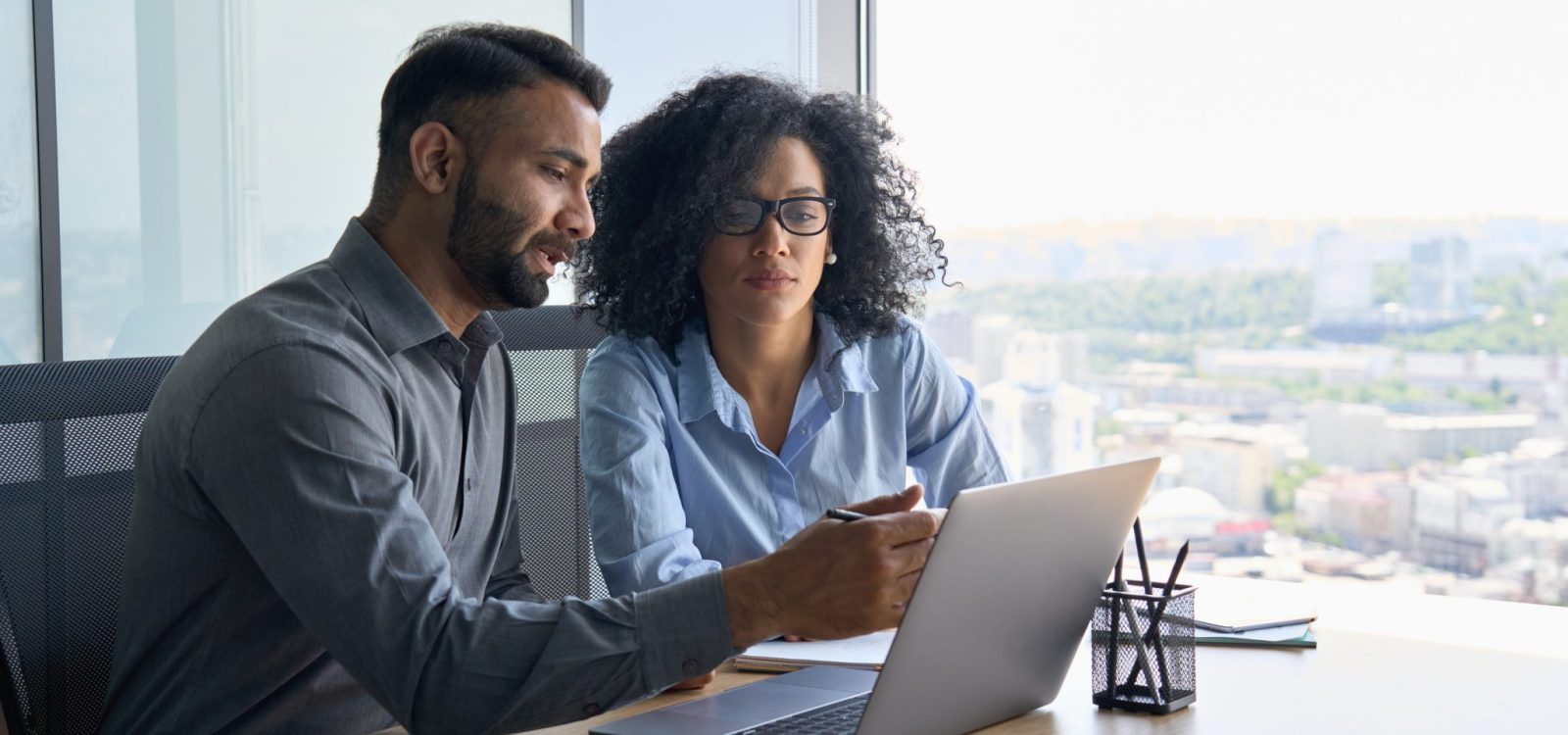
Multi-state Modelling of Customer Transitions
Customer churn is a widespread and expensive problem in general insurance, particularly because contracts are usually short-term and are renewed periodically.
At ICA2023, Yumo Dong and Fei Huang presented on Multi-state Modelling of Customer Churn (Dong et al., 2022). In this article, we delve into their presentation which demonstrated how multi-state customer transition analysis offers deeper insights into how a policyholder’s transition history is associated with their decision-making, whether that be to retain the current set of policies, churn/renew, or add/drop coverage.
Customer churn in insurance
Customer churn (also known as customer attrition, dropout, turnover, or defection) refers to the non-renewal of existing customers for an insurance company.
In general insurance, customer churn poses a major challenge to insurers because contracts are renewed periodically (monthly, quarterly, semi-annually, and annually). With substantial competition and diverse contracts, it is easy for customers to switch insurance providers to satisfy their needs at the time.
Customer churn also poses a challenge in life insurance and other industries such as banking and telecommunications. Keeping a current customer rather than acquiring a new one is often a more profitable strategy for a company, as long-term consumers typically take up less of the company’s resources than new customers.
As such, analyses to determine the drivers and customer behaviours involving churn are critical to a company’s customer retention program. Identifying these customers can help insurers secure continued loyalty.
The break away from traditional customer churn analysis
Traditionally, customer churn analyses have employed models that utilise only a binary outcome (churn or renew) over one period.
However, real business relationships are multi-period, and policyholders may reside and transition between a wider range of states beyond simply churn/renew throughout this relationship. For example, in commercial insurance, an organisation policyholder taking both building and equipment insurance as a bundled contract in Year 1 may decide to drop the equipment insurance but add auto insurance in Year 2, and eventually churn in Year 3. In this case, the policyholder’s state transitions from “building and equipment” in Year 1 to “building and auto” in Year 2, and eventually to “churn” in Year 3; see Figure 1.
The traditional customer churn analysis only focuses on the transition to “churn” in Year 3 but ignores the transition from “building and equipment” to “building and auto” in Year 2. Thus, the traditional customer churn analysis may lead to inaccurate calculation of customer lifetime value and hence non-optimal decision making.
Figure 1: a policyholder’s state transitions from “building and equipment” to “building and auto”, and then to “churn”
Put simply, multi-period data with multi-state transitions more accurately reflects the dynamic changes in the needs of policyholders beyond just churn/renew. For example, the changes in the needs of the policyholder shown in Figure 1 are reflected in the policyholder’s state transitions.
A deeper understanding of the customer needs could secure continued loyalty and ensure sustained profitability. Therefore, there is a strong motivation for insurers to break away from traditional customer churn analysis and take the rich customer transition information into consideration for customer transition modelling.
Multi-state customer churn (transition) analysis
To better encapsulate the richness of policyholder behaviours through time, Dong et al. (2022) propose the multi-state customer churn (transition) analysis. This analysis aims to model policyholder behaviour over a larger number of states (defined by combinations of insurance coverages/contracts taken) and across multiple periods (thereby making use of readily available longitudinal data).
Using multinomial logistic regressions (MLR) with a second-order Markov assumption, Dong et al. (2022) propose a new approach to model the multi-period and multi-state customer transitions, which overcomes the drawbacks of the traditional customer churn model.
With this new approach, which favours interpretability over predictive power, insurance companies can gain a complete picture of how a policyholder’s past states affect their decision to move to the next state, as well as the complex relationships between transition probabilities and various explanatory variables related to premium information, claim information, and contract type. Details of the model building can be found here.
The proposed method is illustrated through an application of multi-state customer churn analysis to a US commercial insurance dataset called Local Government Property Insurance Fund (LGPIF) in Dong et al. (2022). This dataset includes building and content, contractor equipment, and auto insurance contracts. LGPIF is a special line of commercial insurance with its overarching purpose being to make property insurance available for local government units.
However, the proposed multi-state customer churn model can be widely applied to different lines of business. The empirical analysis suggests that:
- A policyholder’s decision on what insurance coverages to buy this year is significantly affected by what insurance coverages the policyholder has bought in the past years. Technically speaking, the second-order states of origin play an important role in distinguishing between different transition probabilities based on the empirical dataset LGPIF. For example, there would be a strong signal to churn when a policyholder moved from full coverage to partial coverage in the past two years (states).
- Premium information, claim experience, and contract information play statistically significant roles in determining policyholders’ transition behaviours beyond simply whether policyholders churn or not e.g adding or dropping coverages. For example, for the policyholders who took all three insurance contracts (building, equipment, and auto) in the last two years, if they experienced larger total premium increases, then they would be more likely to churn than cancel one or two existing contracts this year. The proposed multi-state customer churn model takes these longitudinal transitions into consideration and provides a holistic model to understand and predict customer behaviours, including but not limited to churn.
- Multi-state customer churn analysis generally improves the accuracy of customer lifetime value (CLV) calculations compared to the traditional customer churn model (with only one binary outcome (churn or renew) in one period), because the analysis considers possible transitions among different states over several periods when calculating the CLV. This can help firms predict the CLV accurately by incorporating the potential customer transitions (adding/dropping coverages and churn/renew) at each time and design effective marketing strategies to maintain valuable customers.
- The proposed second-order multinomial logistic regression model shows strong out-of-sample predictive performance compared to traditional customer churn models such as binary logistic regression.
- The proposed second-order multinomial logistic regression model can be extended to higher orders and include more states depending on the size and features of the empirical datasets.
Insurers, like any other business, care about customer churn because it directly impacts their profitability and long-term success. This research contributes to the understanding of policyholders’ transition behaviour beyond just churn/renew across multiple periods by proposing the concept of multi-state customer churn analysis.
Using such analysis, insurance companies can gain a complete picture of how a policyholder’s past states affect their decision to move to the next state, as well as the complex relationships between transition probabilities and various explanatory variables related to premium information, claim information, and contract type.
Future research directions
The multi-state, multi-period modelling used in this paper presents a new approach to churn modelling, and so naturally, there are several variations that warrant further investigation.
First, we have implicitly treated all variables as strictly exogenous, which means that we assume all variables are completely unaffected by the customers’ state transitions in the past, present, and future. For future research, variables can be treated as sequentially exogenous, which indicates that current or future customers’ state transitions would cause variables to change in the future. For example, premium and claim could be treated as sequentially exogenous variables, an approach that may provide insights into contractual moral hazard and adverse selection.
Second, the relationship between a policyholder’s claim experience and transition behaviour is complicated and dependent, as it is when a policyholder makes a claim, she has a first-hand experience of the service provided by the insurer. The claims can also indicate higher future premiums. Hence, policyholders who make claims may decide to drop coverages or churn if they think their future premiums are too high or are dissatisfied with the claim processing service. On the other hand, if policyholders are satisfied with the claim processing service and the reasonable future premiums increase, they may keep renewing their contracts. So how to model the claims experience and customer transition behaviour jointly is an interesting area to investigate.
Furthermore, designing effective customer campaigns, interventions, and incentives that help insurers enhance customer loyalty (for valuable customers) and improve claims experience presents another important avenue for future research.
References
Dong, Y., Frees, E., Huang, F., & Hui, F. (2022). MULTI-STATE MODELLING OF CUSTOMER CHURN. ASTIN Bulletin: The Journal of the IAA, 52(3), 735-764. doi:10.1017/asb.2022.18 Available at SSRN: https://ssrn.com/abstract=4279924 or http://dx.doi.org/10.2139/ssrn.4279924
CPD: Actuaries Institute Members can claim two CPD points for every hour of reading articles on Actuaries Digital.