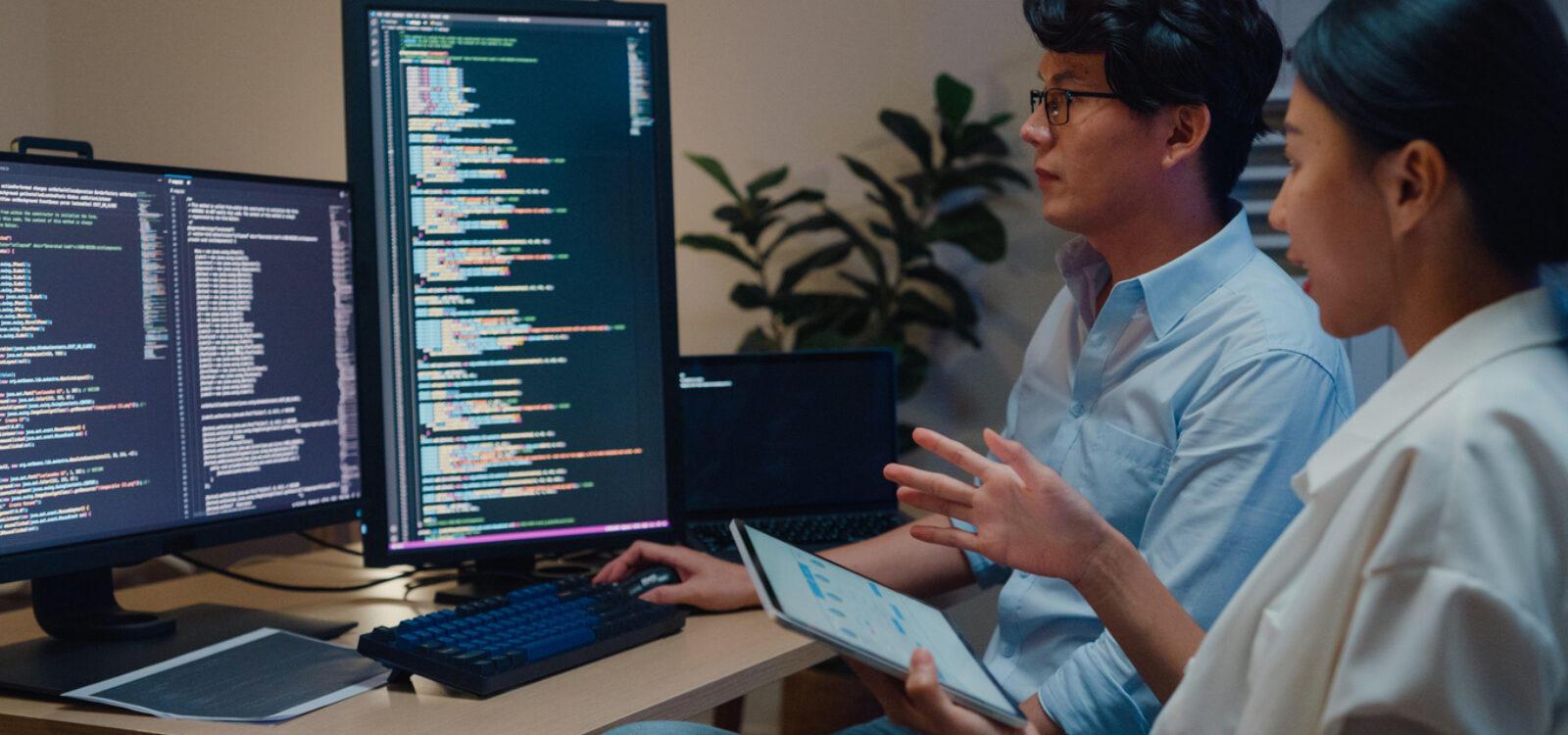
The Unfortunate Preponderance of Edge Cases
In this Normal Deviance column, Hugh looks at the lessons around edge cases in generative AI.
You may have seen the news that Google had to scramble to fix their Gemini AI image generator in late February. At issue were modifications designed to increase diversity when generating images of people.
In many cases, it worked as intended – for example, asking most generators for a group of office executives will produce a more diverse group than the pale and male images that dominate an unmodified generator. However, in the case of Gemini AI, users found cases where the increased diversity is much less appropriate – asking for images of German World War 2 soldiers, or American Founding Fathers, produces images that are historically inaccurate and undoubtedly offensive to some.
Figure 1 – Text-to-image AI models try to build diversity into their image generators. Diversity in male executive hairstyles is less of a priority.
Source: “Three business executives in a meeting room”, Microsoft Designer powered by Dalle3
Figure 2 – Gemini AI’s attempt at diversity struggled when applied to historical contexts.
Source: X/@FrDesouche
The key issue here is that the fix (boosting diversity in image generation of people) runs into trouble in a subset of unanticipated cases – specific historical contexts. These small, often unanticipated subsets are often termed “edge cases”. The story is a reminder that edge cases can be both tricky and important.
Edge cases can be far more common than people appreciate. I’m reminded of the study of body sizes amongst US Air Force pilots in the 1950s that showed that none of the 4,000 pilots had measurements that were in the average range for all dimensions. This led to a radical rethink of how cockpits were built, with an increased focus on adjustability. In that situation, every pilot proved to be an edge case.
Edge Cases in AI
In the world of modern AI, there is a massive proliferation of edge cases. Since text and image generation have ultimate flexibility in what is asked of them, the potential issues to consider are limited only by the creativity of users.
Overcoming this relies on significant amounts of human intervention. In the case of OpenAI, “red teams” are hired to try to produce harmful content from ChatGPT responses, and model training requires extensive human-based tagging of harmful content to try to better filter it out of models. Dealing with edge cases can be time-consuming and expensive.
The potential costs of edge cases can be hard to anticipate. Recently Air Canada was found liable to offer discounted bereavement airfares outside their standard policy due to errors made by the unsupervised ChatBot on the company webpage. I’d be surprised if the interaction between ChatBot and these slightly obscure ticket policies had been extensively tested.
In more traditional actuarial contexts, similar risks attached to edge cases occur
For some flavours of insurance, pricing engines are sufficiently complex that the risk of unforeseen edge cases grows. For example, general insurance premium offers must elegantly handle situations where a person wants to maximise their sums insured, set a very large excess, or request other specific policy inclusions and exclusions. A model that handles the extremes poorly can end up pricing too low (adverse selection) or too high (customers forgone).
Similarly, for capital and reserving contexts, disproportionate amounts of time must be spent on extreme risks, very large claims, and hard-to-quantify systemic risks, all of which tend to sit on the edge of a reserving model and historical experience.
What can be done?
In some cases, there may be a need to be more selective in where we choose to implement complexity. Simplifying an offering such as reducing to a smaller set of rating factors reduces the chance of unexamined edge cases cropping up.
Where we do pursue complexity, new models and technologies need robust testing and validation, where people with a strong understanding of both the model and broader context explore situations where they break down. This is an inevitable additional cost to consider when rolling out a model.
Aside from that, as the use of AI models grows, we will have to become more accustomed to living on the edge.
CPD: Actuaries Institute Members can claim two CPD points for every hour of reading articles on Actuaries Digital.