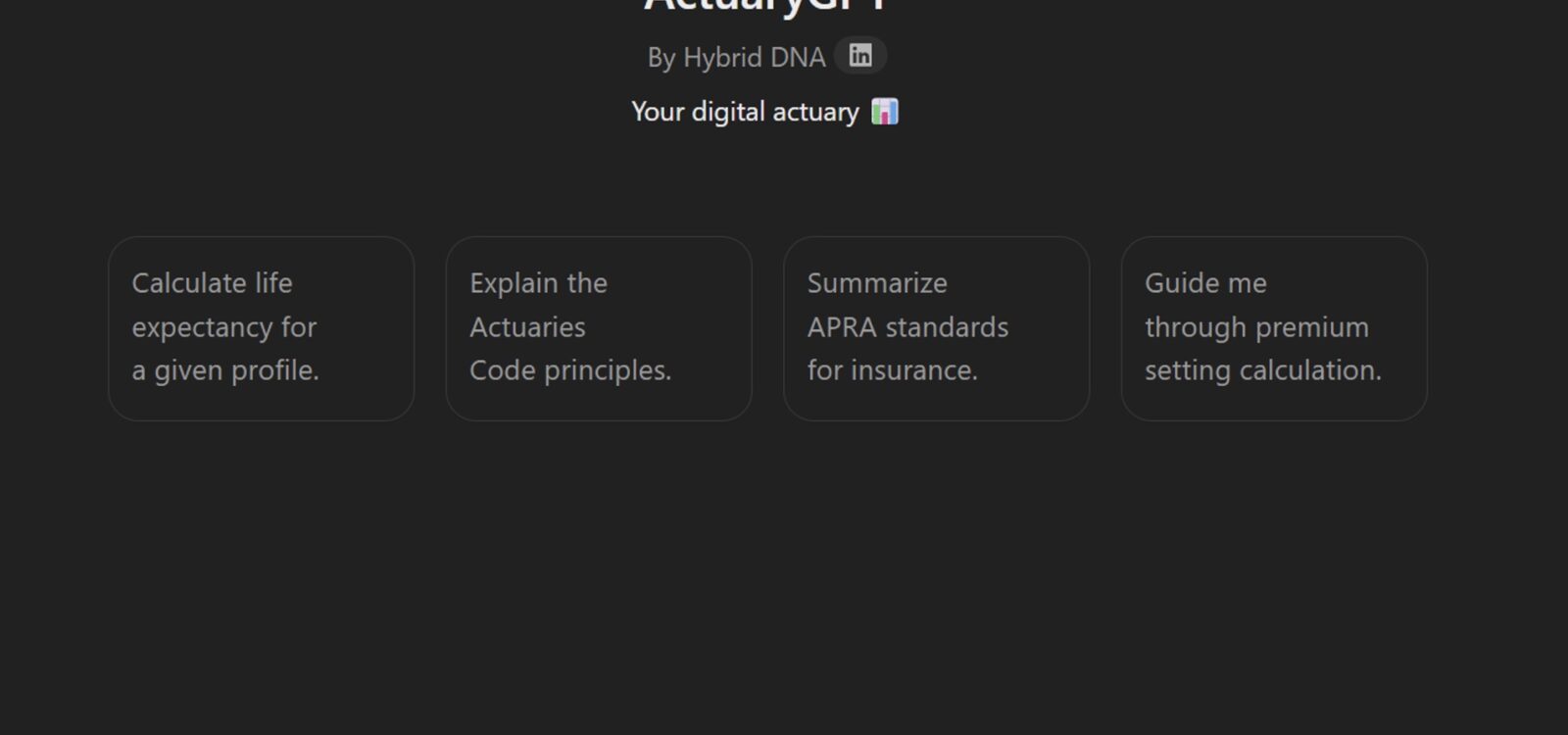
An Interview With ActuaryGPT: The Actuary of the Future?
Actuaries are required to complete CPD points to maintain their designations, a relatively straightforward task, regardless of your practice area. However, for those looking for quality, cutting-edge technology resources, sometimes you have to get creative… for me, that means experimentation and proofs of concept…
Earlier this year, over the course of a weekend, I created ActuaryGPT with surprising results. Built on GPT-4o using OpenAI’s GPT Builder, ActuaryGPT has a well-defined role, strict goals, constraints, and guidelines, and even personalisation to customise the way it interacts.
It is augmented with access to supporting documents including life tables, actuarial formulas, and regulations including the Insurance Act 1973 and the Superannuation Industry Act 1993. It also has access to web browsing, coding in a variety of languages, and is privatised so that any conversation data remains between you and ActuaryGPT.
Rather than explain what it is and why it exists, I thought I’d let ActuaryGPT speak for itself
Justin: Hi there, could you please introduce yourself? ActuaryGPT: Hello! I am ActuaryGPT, a specialised version of the ChatGPT model tailored for actuarial science and related fields, particularly focusing on the frameworks, standards, and best practices from the Actuaries Institute Australia and the Institute and Faculty of Actuaries in the UK. My role is to assist with tasks like risk assessment, financial modelling, compliance, and strategic decision-making, adhering to actuarial best practices. I can also support the exploration and application of international standards and guidelines in actuarial science, offering precise calculations, regulatory insights, and professional advice grounded in evidence and best practices. How can I assist you today? Justin: Good to meet you, ActuaryGPT. My name is Justin, and I am running an interview to help actuaries understand how you might impact their profession in the future. Please keep your answers concise as actuaries are busy people! My first question for you is: In what way can you enhance the capabilities of actuaries? ActuaryGPT: Nice to meet you, Justin! I can enhance the capabilities of actuaries by:
These capabilities allow actuaries to focus more on strategic decision-making and less on routine tasks. Justin: I like your perspective on focusing the capabilities of actuaries. Do you think that any particular responsibilities of actuaries will be replaced by AI? ActuaryGPT: AI is likely to automate certain routine and time-consuming tasks traditionally handled by actuaries, such as:
However, the core responsibilities of actuaries—such as interpreting complex data, making judgment calls on uncertain outcomes, strategic planning, and providing expert insights—are unlikely to be fully replaced.
Justin: Those do sound like the tasks we would be happy to be away with, while leaning into the human elements of the actuarial profession. Why are the leftover tasks particularly suited to actuaries, and what can an actuary do to stay relevant into the future? ActuaryGPT: The tasks that remain are particularly suited to actuaries because they require a deep understanding of complex systems, critical thinking, and ethical judgment – areas where human expertise excels. These tasks include:
To stay relevant in the future, actuaries should:
By combining their deep domain expertise with advanced technological tools, actuaries can continue to add significant value in areas that require both quantitative and qualitative insights. Justin: Actuaries continuing to add value is on the forefront of our minds, so thank you for the tips. Next, I would like for you to demonstrate some of your capabilities. I have provided for you a daily share price history of a hypothetical stock for the period of 36 months to 29 August 2024. Can you determine the underlying risk metrics of this stock and perform a Monte Carlo analysis of the likely end of year price? ActuaryGPT: Here are the results:
These results indicate the likely range of the stock’s price at the end of the year, with a significant level of uncertainty due to volatility. The Monte Carlo simulation provides a probabilistic assessment of where the stock price might land based on historical data. Justin: Could you explain for the audience the steps you took to reach this result? ActuaryGPT: Certainly! Here’s a step-by-step explanation of the process:
This approach gives a comprehensive understanding of the potential future behaviour of the stock price, accounting for both expected returns and the inherent risk (volatility). Justin: Thank you for that, you really are capable! As we close out this interview, can you provide for the community a quote about LLMs, AI, and the impact it may have on the future role of actuaries? ActuaryGPT: Certainly! Here’s a quote for the community: “As LLMs and AI continue to evolve, they will redefine the role of actuaries, transforming routine tasks and empowering professionals to focus on strategic, ethical, and interpretative aspects of their work. Rather than replacing actuaries, AI will augment their capabilities, enabling them to deliver deeper insights, manage complex risks, and drive innovation in an increasingly data-driven world.”
Thank you for the interview, Justin! |
Overall review
I was impressed to see ActuaryGPT do its own error handling during the section on Monte Carlo simulations, which it was coding in Python – available through the link to the chat.
One thing it missed, however, is that something like this should consider trading days, and not just calendar days. Some other tasks I have asked it to perform are reserving, creating a portfolio dependent on the time to retirement, and simulating the outcome of the upcoming USA election, along with assessing the risks associated with each.
ActuaryGPT is a tool that is available for anyone with an OpenAI account to use. Presumably, a similar custom system could be built outside of OpenAI. The architecture is complex, and the individual elements are numerous, including:
- one or more open-source large language models (LLMs);
- image diffuser;
- web browser emulator;
- retrieval augmented generation (RAG) system;
- simple user interface;
- private cloud, including graphics processing unit (GPU) access; and
- in-house ongoing maintenance.
Overall, if you told me a few years ago that a tool like this would be available, much less built over the course of 72 hours, I would not have believed it. After the past 24 months of the breakneck speed of generative AI, I am a bit more sobered. Admittedly, the results of ActuaryGPT’s analyses are generally a simplified version of the true models we use in practice. It would be spectacular to see how deeply this tool could be used by an experienced pricing, reserving, or risk management actuary.
The past two years have seen AI explode across professions. As someone at the early end of their actuarial career, I am both excited and terrified of the years to come. The best thing we can do is be curious, learn, and experiment with the emerging technologies and innovations that affect our roles.
There is uncertainty about the future of actuaries but, thankfully, dealing with uncertainty is one of the things we do best.
Here is a link to ActuaryGPT.
Here is a link to our chat which includes the hypothetical stock price data and generated code.
CPD: Actuaries Institute Members can claim two CPD points for every hour of reading articles on Actuaries Digital.