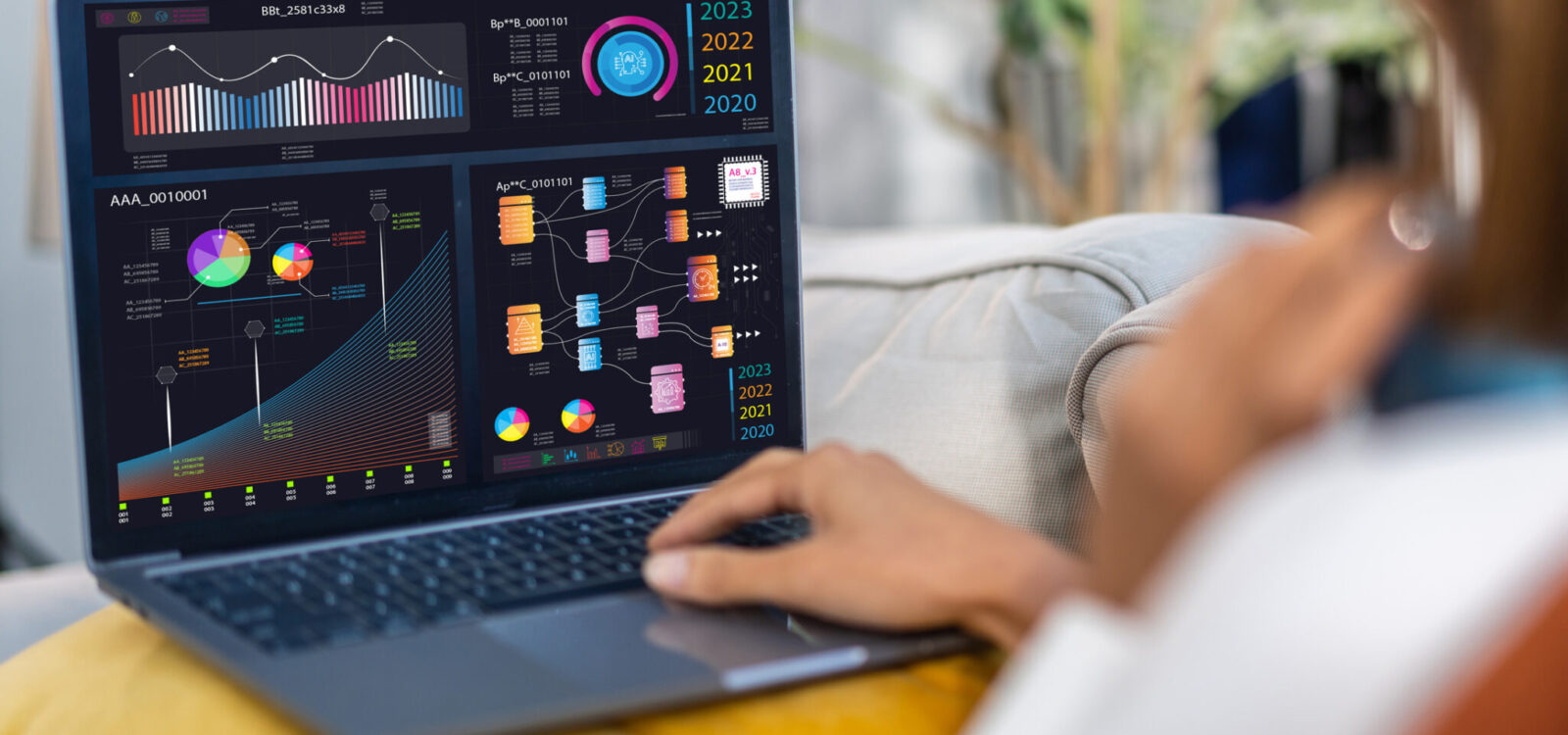
Visualising Data
The sheer volume of data produced daily is staggering[1], and without proper interpretation, it remains just numbers.
This is where data visualisation comes in – transforming data into formats that can be easily understood and utilised.
Research consistently shows that humans better understand and retain information presented in charts rather than raw numbers.
For instance, a study[2] found that people remember data more accurately and for longer periods when it is presented visually rather than textually.
Throughout history, this principle has been evident. From ancient cave paintings depicting hunts to Florence Nightingale’s groundbreaking use of polar area diagrams to illustrate mortality rates during the Crimean War. Visuals have been a powerful tool for storytelling and information dissemination.
Today, data visualisation remains a cornerstone of how we consume data.
The most important step
It might come as a surprise to many that the most crucial step in data visualisation is not the actual process of turning tables into charts. Rather, it is understanding the context in which the data will be presented as context is king. Without context, even the most beautifully crafted visualisations can fall flat or worse, mislead.
Understanding the audience, the message you want to convey and the action you hope to inspire, are all fundamental considerations before any visualisation work begins.
Choosing a visual
There are over 100 different ways to visualise data, according to experts like Stephen Few and sources such as The Data Visualisation Catalogue[3].
However, most people tend to use a core set of five types of visuals. Each of these has its unique strengths and use cases.
Source: Stockland. (2023). Annual report for the year ended 30 June 2023. Stockland. https://www.stockland.com.au/globalassets/corporate/investor-centre/fy23/fy23/stockland-annual-report-30-june-2023.pdf
Text might seem counterintuitive as a ‘visual’ but it can be very useful if you have just a number or two to highlight.
Make the numbers as prominent as possible with a few supporting elements to enhance the message. These are often seen in company financial reports as key results.
Source: Knaflic, C. N. (2020, September 24). What is a table? Storytelling with Data. https://www.storytellingwithdata.com/blog/2020/9/24/what-is-a-table
Continuing with the theme of counter-intuitiveness, tables are useful for communicating multiple units of measure to a mixed audience, each focusing on their area of interest.
Tables engage the verbal system, meaning people tend to read them. It’s important that the table design doesn’t overshadow the data.
A variation of a table is a heat map, which uses colour saturation to reduce mental load and help users quickly identify high or low values.
Points are great for showing relationships between two variables because they encode both data points (and more with the sizing) simultaneously on the axes.
They tend to be used in technical fields and can sometimes be perceived as complicated to understand.
Lines are usually used for plotting continuous data, often represented in some unit of time.
Because of the implicit assumption of a connection between points, line graphs are usually good for identifying trends.
Note that when graphing time, the intervals between points should be consistent to avoid misrepresentation.
Source: Knaflic, C. N. (2020, February 19). What is a bar chart? Storytelling with Data. https://www.storytellingwithdata.com/blog/2020/2/19/what-is-a-bar-chart
Bars are a common choice for data representation, often seen as both boring and easy to recognise.
Our eyes compare the ends of the bars, making it simple to identify the largest, smallest, and incremental differences. Always use a zero baseline to avoid misinterpretation.
Variations such as horizontal bar charts and waterfall charts are frequently used in corporate settings.
Visuals I Avoid
Pies and Donuts generally do not work well because people are less good at quantifying area by comparing angles or looking at a 2-dimensional space.
Where this is negated either by big differences in the categories, or labelling the portions, there is generally a better option to represent the results.
Source: Knaflic, C. N. (2020, May 14). What is a pie chart? Storytelling with Data. https://www.storytellingwithdata.com/blog/2020/5/14/what-is-a-pie-chart
3D Charts introduce unnecessary complexity into the visual unless you’re trying to plot a 3rd dimension.
Source: EDUCBA. (n.d.). 3D plot in Excel. EDUCBA. https://www.educba.com/3d-plot-in-excel/
Even if that’s the case, you might want to reconsider visualising the data in another way that would fit a flat representation, as it can be very tricky to navigate.
Once we’ve chosen an appropriate visual, the next step is having a process to turn our default application themes into easy-to-understand concise visuals.
Reducing clutter
Creating a chart often begins with a noisy and cluttered default. An overly cluttered chart may lead to unnecessary cognitive loads for the audience and so can hinder the decision-making process.
Most people already go through some process of reducing clutter by adjusting the chart and making it ‘prettier’. There are many ways to go about doing this but I find the gestalt principles of visual perception a useful framework.
Source: Nastenko, M. (n.d.). Gestalt principles in data visualization. Medium. https://nastengraph.medium.com/gestalt-principles-in-data-visualization-a4e56e6074b5
Proximity: Chart elements should be placed near related elements.
Similarity: Colours should be used to highlight similar characteristics.
Enclosure: Group related elements with the same background and highlight specific parts of a chart that might be important.
Closure: Eliminate unnecessary elements and borders in a chart.
Continuity: Arrange elements to reflect how our eyes would read the chart, for example, top to bottom, left to right.
Connection: If there’s a connection between two datapoints, join them up with a line.
As the theory can sometimes be abstract, below is a concrete example of how to apply several of the principles to declutter a simple line graph representing the monthly claim counts each year for a fictional insurer.
The chart reflects a very common default template for a line graph created in Excel. While this is an okay visual, there’re ways we can reduce clutter and thus, the cognitive load for the reader.
Although the effect might not be as obvious here, removing chart borders is usually a first good step in line with the principle of closure. Whitespace can be a good alternative to differentiate the visual from other elements on the page if required.
In some cases, it might be helpful to keep the gridlines, but we’ll remove it here as that is not the key point in the chart. If you do need to keep the gridlines, make them thin and use a light colour to avoid letting them compete visually with the data.
We further reduce the cognitive load here by removing the markers. These do not add any informational value and are already represented by the combination of lines and axis labels.
Markers might be useful but use them deliberately as opposed to an application’s default setting.
Trailing zeroes on the y-axis here (and in most cases) do not carry any value and can be removed. Given that this chart is all within a year, we’ve also moved that information partially into the title, thereby making the x-axis a lot easier to read.
While legends are nice, we can make use of the proximity principle to put data labels next to the data they describe without the extra element.
Using the principle of similarity to make the data labels the same colour as the data they describe further enforces the idea to the audience that the two pieces of information are related. We can also remove duplicated information in the title and put it on the top left using the rule of continuity.
While the chart is incomplete, the adjustments made so far have reduced the number of elements that did not provide informational value to the overall graph.
Insurance examples
In the insurance industry, certain methods for representing results and reporting are widely utilised across various lines. Below, we provide examples demonstrating the application of these principles noting that all figures in the following charts are fictional.
A common use case involves presenting trends and performance by rating factors. Combined charts are frequently used to depict both exposures and observed measures simultaneously.
Among the principles at work, using colour similarity effectively groups elements together, minimising the need for excessive labelling. This approach also helps to deemphasise the exposure bars, presenting them as supplementary information rather than primary results.
Regular monitoring of sales performance can be effectively achieved without overloading the visual with too many elements. Typically, such a chart requires only the depiction of trends and a quick overview of any shortfalls.
Competitive positioning views can be highly impactful with the strategic use of colour encoding. If the dataset becomes too large, consider grouping subsets of the less significant players to reduce visual clutter. Customer segmentation and strategic decision-making can benefit greatly from scatter plots, with different segments leading to clear and distinct decisions.
The example below uses travel insurance and a sample bubble chart to drive marketing and pricing decisions based on different policyholder segments.
These examples are not intended to serve as strict guidelines. Rather, readers should apply the underlying principles and tailor the design and formatting to their specific business use cases.
A SCOR case study
SCOR hosted its Viz Games around June 2023 in collaboration with Tableau, focusing on designing infographics around the theme of sustainability.
The event highlighted the power of data visualisation in addressing critical issues like environmental impact and sustainability goals. The finalists’ dashboards stood out for their innovative use of visual elements and effective communication of complex data.
These dashboards demonstrate the application of gestalt principles, creating clear and compelling stories that engage viewers. Below are the finalists’ dashboards:
Australia: SCOR Viz Games 2023 – The Inside Scoop | Tableau Public
France: NetZero_by_Sara | Tableau Public
USA: Net Zero | Tableau Public
China: Not a Zero Sum Game | Tableau Public
Each of these dashboards exemplifies the effective use of data visualisation techniques to promote sustainability, making complex information accessible and actionable. They serve as excellent examples of how well-designed visuals can drive understanding and inspire change. Can you spot how many of the principles were being utilised in each of these visuals?
Authors note
All examples provided in the article utilise native Excel functionality for charting.
For a more in-depth read into data visualisation, I highly recommend reading Storytelling with Data[4], which is (in my humble opinion) one of the best books on visualisation I have read and is also the premise for a lot of the content here.
References
[1] Exploding Topics. (n.d.). How much data is created every day? Exploding Topics. https://explodingtopics.com/blog/data-generated-per-da
[2] Berinato, S. (2021, April). How to tell a story with data. Harvard Business Review. https://hbr.org/2021/04/how-to-tell-a-story-with-data
[3] DataViz Catalogue. (n.d.). Data visualization catalogue. https://datavizcatalogue.com/
[4] Knaflic, C. N. (n.d.). Books. Storytelling with Data. https://www.storytellingwithdata.com/books
CPD: Actuaries Institute Members can claim two CPD points for every hour of reading articles on Actuaries Digital.