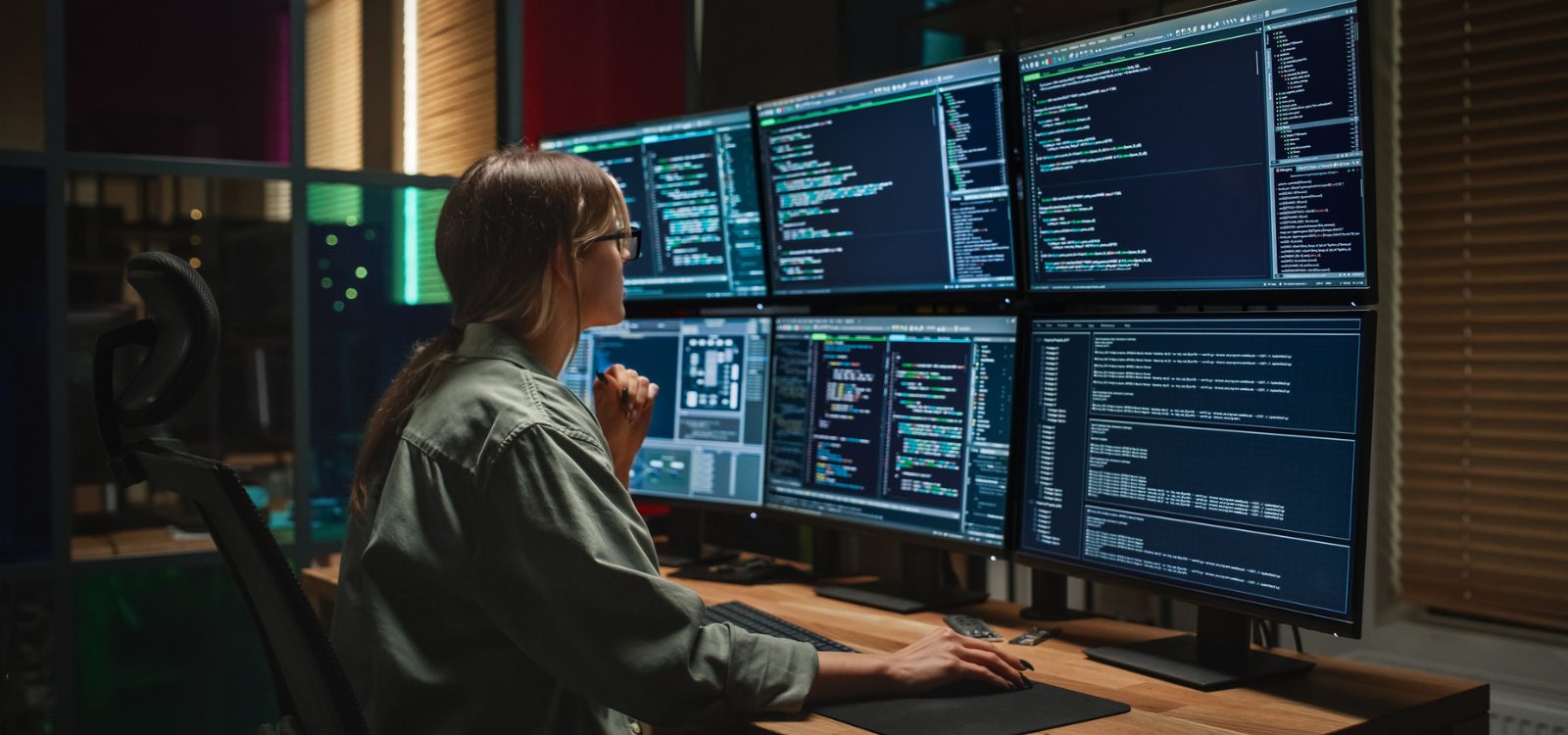
How can model governance capture value from AI in insurance?
Simon Lim reflects on the use of AI in insurance and suggests a practical framework for assessing AI models and managing risks.
Summary
- Artificial intelligence and machine learning are prediction machines that are increasingly being deployed in insurance
- These models can predict more accurately but explaining them is harder due to their complexity
- Governance is essential: Insurance leaders are still accountable for the risks arising out of AI usage
- Clarify the value of AI using a three-stage process:
- Technical quality: How accurately the model predicts
- Explainability: Whether the predictions are understood and whether the business trusts the predictions
- Business value: Is the model value for money?
- Sequence the evaluation in increasing order of human supervision to optimise speed and cost
- AI model governance requires oversight by multi-disciplinary professionals with insurance domain knowledge
- Optimise the governance process through appropriate team structuring and model infrastructure.
Introduction
Major Australian general insurers are investing heavily in deploying artificial intelligence (AI) and machine learning (ML) models. This trend is likely to accelerate as the tremendous value of AI becomes widely known.
The term artificial intelligence (AI) commonly refers to specialised predictive machines, i.e. software/algorithms that take data as input, and output predictions. Although AI also includes other areas such as unsupervised learning, predictive algorithms are the focus of this article. In insurance pricing, predictions are focused on the expected claims cost of a policy. There are many different types of AI models, with machine learning being one of them and the focus of this article.
Traditional insurance models, such as generalised linear models (GLMs), also predict the claims cost. The key benefits of more advanced AI (or machine learning) models are:
- AI models can be more accurate (subject to data quality)
- They allow insights to be derived from larger and more complex datasets
- They may cost less to operate because they can be more quickly updated. Although they can technically be fully automated, given the complexities of insurance and its data, human supervision is an essential component of governance.
Machine learning (ML) models are harder to evaluate and explain because they are more complex and automated. Specifically:
- They are typically composed of hundreds of sub-models (such as folds, nodes or trees)
- Larger and more complex datasets are typically used
- The relationships between data and predictions derived by each sub-model are themselves more complex (non-linear) and automatically selected by an algorithm.
Explaining AI is like interviewing a candidate for a job.
An interesting point to note is that explaining an AI model is generally done top-down, such as running the model under different conditions and seeing how it changes or building a simpler model that emulates the AI model.
In contrast, explaining a GLM can be done bottom-up where its underlying mechanics are interpreted directly. AI explainability is like interviewing a candidate for a job. The candidate is assessed via a discussion which involves asking hypothetical questions, but the interviewer is not able to observe on-the-job performance firsthand.
The need for AI model governance
Despite this complexity, insurance leaders are still accountable for the accuracy and risks arising out of AI usage. Competition will likely force businesses to implement AI. However, AI introduces new risks, such as whether the promised benefits are realised within budget and reputational risks associated with misuse or perceived misuse of data and AI.
As leaders are accountable for these outcomes, an understanding of how to evaluate AI models and how to ensure they meet business needs is therefore essential.
A staged governance framework that optimises speed and cost
AI model development follows a process like manufacturing. Code is written to build a “machine” and data is passed through this “machine” to produce predictions. Checks should be performed throughout this process and development iterated to minimise re-work.
We propose an efficient model governance framework which sequences activities in increasing order of required supervision and cost, as shown in the figure below.
Checks that are systematised and low cost, such as statistical tests, should be applied first prior to more complex assessments. This sequence increases the rate of development and model effectiveness because:
- Early stages of the model can be checked early and more frequently, before further work is invested
- The available time for human judgement can be focused on more refined models, saving labour
- Iterations and learnings are obtained at a faster rate.
The three stages of the model governance framework are as follows.
1. Accuracy: How well does the model predict?”
The key goal at this stage is to apply quantitative metrics which may indicate problems with the model such as over-fitting or poor accuracy.
These statistical tests and interpretations can be typically systematised and are therefore low cost to run once established.
Typical tools to use include: GINI coefficient, Goodness of fit metrics, cross-validation, one-way charts and actual versus expected heatmaps.
2. Explainability: Do the predictions make sense? Do we trust it?
Lack of explainability, consistency or intuitiveness may indicate (although not guarantee) that the model has fitted to noise and may perform poorly in a live environment.
Although metrics used in these tests can also be systematically run, interpretations require judgement and an understanding of context and therefore full automation is generally not possible.
Common queries made in this stage include:
- Are the key drivers consistent across each sub-model?
- What are the trends across continuous variables?
- Is there sufficient data backing the relationships derived by the model?
- Are there any odd features of the model?
- How can model development be improved in light of these results?
Typical tools to use include: Partial Dependence Plots (PDP), Feature Importance, Shapley plots and dependence plots, one-way plots.
3. Business value: Is AI value for money?
Once a model has passed stages 1 and 2, it is technically sound and the business has confidence that it will perform in a live environment. However, the value of the AI model should be quantified in order to justify the model’s deployment cost.
It is vitally important that business objectives are clear. According to McKinsey[1], “unclear objectives” and “lack of business focus” are the top issues driving IT cost overruns and failures.
The two use cases for increased modelling accuracy are in being able to more effectively:
- Avoid or re-price less profitable risks
- Optimise volumes and grow in profitable segments
The true value of the model lies in the increased profits or superior business outcomes arising out of these strategies. This can be quantified via scenario and price elasticity analysis.
For example, for the risk avoidance case, we could quantify the loss ratio improvement if the 2% worst risks identified in a backtest were avoided or re-priced. For the growth scenario, we could quantify the increased profits if the business were to growth in a selected highly profitable segment.
There are some practical considerations when quantifying such scenarios. Firstly, any price increase scenarios should adjust for loss ratio improvements purely from higher prices. Secondly, the relationship between price and volume, i.e. price elasticity, should be incorporated and such assumptions agreed with stakeholders.
Like any investment decision, this test is judgemental due to the large number of possible scenarios. Nevertheless, it is important to ensure the business derives value from AI.
AI still needs humans…for now
Model governance is not a mechanical process. It requires supervision by people with technical expertise and commercial judgement in addition to insurance domain knowledge.
Model governance is also labour intensive. We suggest some strategies to ensure governance does not become too burdensome:
- Evaluate on the fly: Evaluate and build iteratively with milestone gateways. This means evaluating while building and providing feedback to the development team as they occur.
- Fast feedback means those building models do not waste time on less useful paths.
- Appropriate technical infrastructure: Build the necessary infrastructure that can to run tests quickly. It will be inevitable that tests are run and re-run throughout the iterative process. Invest the necessary resources upfront.
- Trade-off flexibility and cost: Build infrastructure that is flexible but trade it off against cost and time. A model infrastructure that is static and hard to change is not ideal, but neither is one that is very flexible but requires massive investment to set up. In some situations, an ad-hoc approach is best, while in others it is not.
- Appropriate team structure: There are many team structures that can deliver effective model governance, however in my experience, model evaluation should be a distinct responsibility that is just as important as model development. Team members need to be given enough time to run evaluation metrics at the required level of detail and the team leader needs to be accountable for governance outcomes.
Until a new generation of AI emerges that can self-review effectively, AI governance will continue to be essential in order for businesses to manage risks and derive value from AI.
[1] Source: KPMG, Credit Suisse, Allianz, NfX, Customer Monitor
[2] https://www.reuters.com/article/us-amazon-com-jobs-automation-insight/amazon-scraps-secret-ai-recruiting-tool-that-showed-bias-against-women-idUSKCN1MK08G
[3] https://www.mckinsey.com/business-functions/digital-mckinsey/our-insights/delivering-large-scale-it-projects-on-time-on-budget-and-on-value
CPD: Actuaries Institute Members can claim two CPD points for every hour of reading articles on Actuaries Digital.