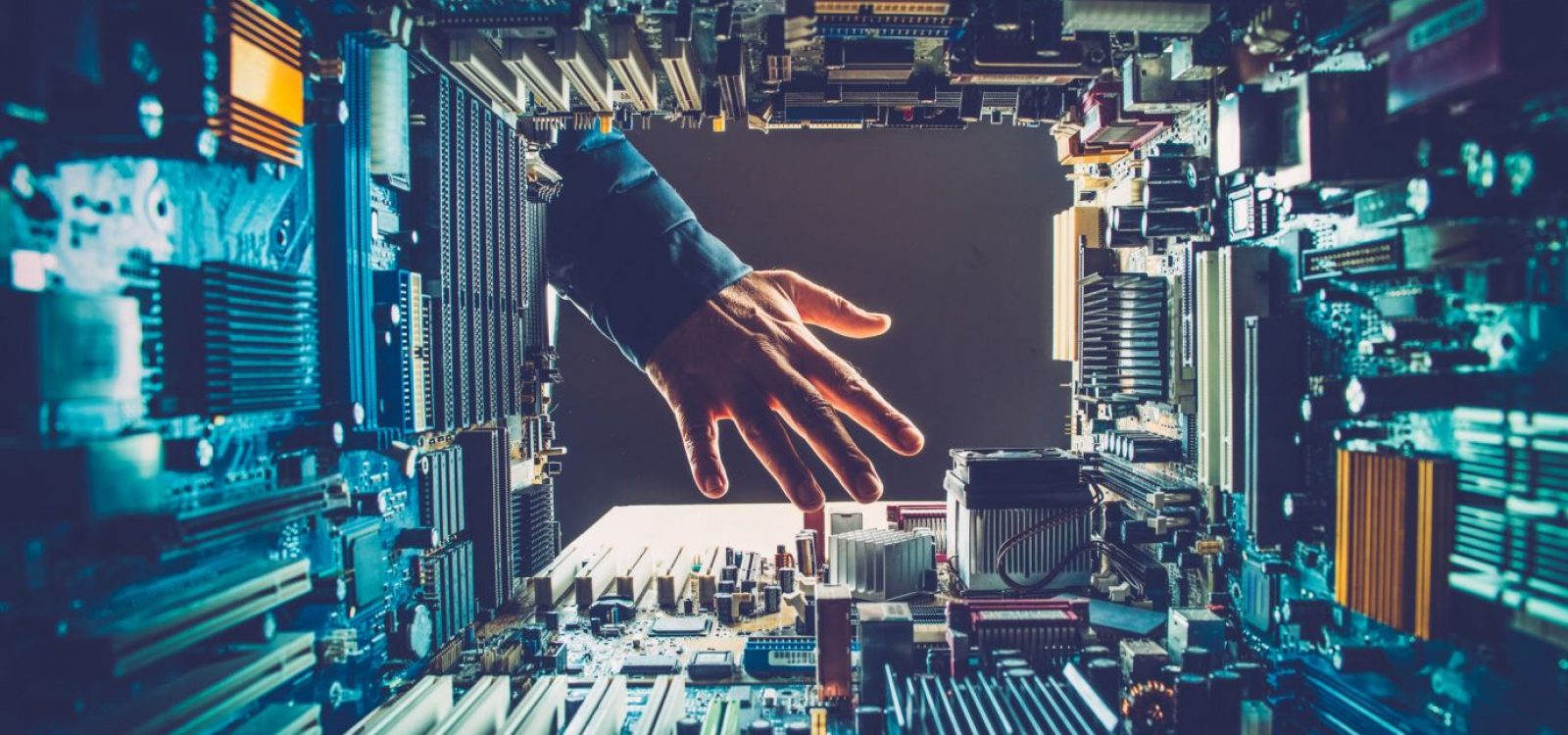
Normal Deviance – Seeing the forest for the analytics trees
Our models are often built on the high-quality data that we can see, but ignore valuable information that falls outside our usual data collection. Hugh unpacks this idea in his semi-regular Normal Deviance column.
Recently I was invited to a meeting of the Collaborative Partnership, which aims to improve work participation for people with physical or mental health conditions, by taking a cross-system view. There are some good resources there if you are interested in understanding the ways that different supports are given (through workers’ compensation, insurance and welfare).
More broadly, the experience reminded me that analytics work is often very good at optimising the ‘known universe’ and often incredibly poor at providing insight outside that universe. For example, in workers’ compensation, a large amount of detailed modelling has been done to understand how injured workers move through the compensation scheme, but there is much less known about what happens once their compensation payments stop. Do they return to work? Or drop out of the workforce and not return? Do they have extended spells on welfare benefits? Or rely on insurance payouts?
Analytics work is often very good at optimising the ‘known universe’ and often incredibly poor at providing insight outside that universe.
This type of knowledge gap occurs in all sorts of analytics contexts. For instance:
- Significant time and effort is now spent on monitoring sentiment from social media, which allows rich detail and insight to be generated on an interesting (but ultimately not entirely representative) portion of the population. Views beyond social media, which are harder to monitor, can be neglected. The gap between digital and non-digital is a consistent area where analytics models are incomplete; digital advertising is likewise a more measurable than traditional advertising spend but usually provides an incomplete picture of the customer base.
- Insurers can have sophisticated models for certain sales channels (e.g. powerful demand estimates for online quotations) but have relatively crude approaches to other channels. For many years, group life insurance suffered in this fashion. It represented a significant portion of an insurer’s policies but had many unknowns and limited ability for the insurer to understand and manage their underwriting and risk profile.
- Banks can be incredibly detailed in their understanding of a customer, to the extent that the customer’s accounts, credit cards and loans are bundled with the one bank. But a customer will often have accounts or products with other banks too, leaving a major hole in the understanding of a customer.
- Government programs (such as housing programs) often cater to a particular cohort of people, for which the characteristics and needs can be well understood. But there will also be people just outside the eligibility of a program who have similar needs, which are sometimes met in other ways. This ‘latent demand’ is potentially invisible to government without significant work.
Such myopia creates an obvious opportunity to investigate the ‘known unknowns’. While these are, by definition, harder to get right, there are some useful ideas that can help.
1. A simple but robust answer to a big question can be more useful than a detailed answer to a small question
Often there are ways to attack the bigger question, for example through using targeted surveys or conducting other research. Such evidence will not be as cutting edge as detailed modelling of the ‘known’ systems, but will enable broader and better questions to be answered. This type of thinking may affect how analytics projects are prioritised.
2. Partnerships are possible
In government, increasing use of data linkage is improving our understanding of how people move between systems, or potentially fall through the cracks. For corporates, data partnerships are possible to address similar sorts of cross-system questions, assuming appropriate privacy safeguards and consumer communication are in place. Vertical integration for companies can also achieve a broader customer view.
3. The biggest risks can lie outside the system
In the banking example given above, the risk of a customer leaving is significantly higher if they have accounts with other providers. A churn model that does not attempt to explore this risk is missing a trick.
4. Models are getting better
While the true status of a customer outside known datasets may be unknowable, it can often be inferred probabilistically from what is known. In such cases, it’s possible to meaningfully talk about the entire population despite the narrower scope of a dataset. For example, mortality rates attached to group life insurance must still be a legitimate subgroup of population-wide mortality, which is well-understood.
We may never completely solve cross-system gaps. However, by thinking through how pieces fit together, we can reduce the risk that a model carefully built on main company databases becomes quickly outdated as the external environment evolves.
CPD: Actuaries Institute Members can claim two CPD points for every hour of reading articles on Actuaries Digital.