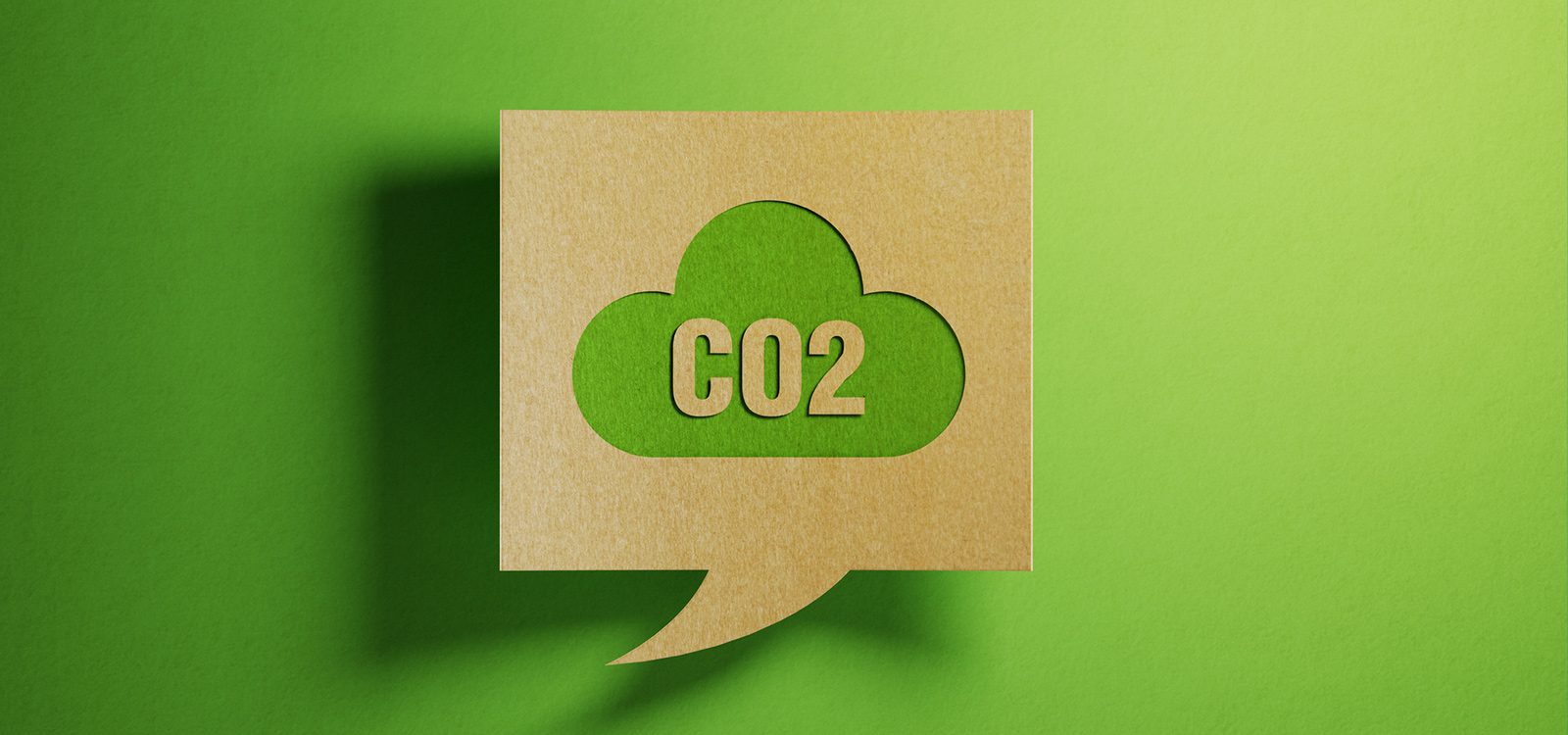
Can machine learning optimise the carbon capture process?
In the last two decades, carbon capture (CC) has emerged as an essential technology for mitigating emissions from power plants and other industrial processes. Yet, many environmentalists and scientists criticise it, saying it does more harm than good.
Carbon capture is the process of removing CO2 from the atmosphere through direct air capture. Once captured, there are two options; It can be permanently stored underground or used to produce products requiring carbon such as fuels or specialty chemicals.
Many believe that this is a vital technology to combat the impacts of the burning of fossil fuels, which continues to contribute to the deterioration of the environment and accelerate climate change.
In theory, this sounds like a no brainer – we have released too much carbon into the atmosphere, so let’s capture it and put it back. However, in practice, it has not been so successful. Optimising the CC process is extremely costly from a time, money, and energy perspective. Many plants have a net negative effect – polluting more than they capture.
The only so-called ‘working’ plant in Australia is in the north-west of WA, managed by the energy company Chevron. According to their agreement, the plant was meant to be capturing and storing 4 million tonnes per year from 2016. Whilst the plant momentarily worked in 2019, this failed attempt has captured only 30% of what it was supposed to[1] as well as emitted 10 million tonnes of CO2. For context, 10 million tonnes is the equivalent to the size of emissions of every single flight taken in Australia yearly (pre-COVID).
So, the question arises: How can CC be improved to be made more efficient and minimise the cost to the environment? The answer I propose is through machine learning.
Prior to machine learning, the traditional mechanistic-based methods of modelling and optimising the carbon capture process relied heavily on an extensive knowledge of the underlying scientific laws. Optimisation is sought through an immense amount of physical experimentation and iteration. Furthermore, the success of capture varies significantly by environmental conditions. For example, a CC plant in Australia looking at the techniques and methods of a successful plant in Canada would be deemed useless, as the chemical environment is vastly different. So, not only does this iterative optimisation process use up a lot of time and money, but it also emits carbon itself.
By taking a data-driven approach, a significant portion of the physical experimentation can be taken out, as well as its associated emissions.
There are generally two goals of a machine learning model in the carbon capture process:
- Predict the thermodynamic properties to absorb CO2. In other words, what chemical environment will best capture the CO2.
- Optimise the capture rate. In other words, minimising the energy penalty, whilst maximising the amount of CO2.
A study conducted at the University of Regina, Canada, had a CC system dataset, and was aiming to understand the predictor and predicted parameters in the CO2 capture process.
Their process of model selection was as follows. They first started with a simple statistical regression, due to its ease in application and interpretability. However, a statistical regression does not explain irregular/non-linear relationships, which are likely to exist among the parameters in the capture process. Next, they tested a neural network combined with a sensitivity analysis. Whilst this built on the statistical analysis and allowed for non-linearity, the models are opaque and cannot explain the nature of the relationships between the parameters. This is quite important in the CC application as it is desired to understand relationships, such that it can be validated from a scientific perspective.
Building on this, they tested an adaptive neuro fuzzy inference system model. It could accurately model the irregular/non-linear relationships, and it could explain the relationship between the input and output variables. Although, it could only generate ‘fuzzy rules’ which require the parameters to be subdivided into categories such as high medium low. Also, the number of rules generated was the number of input spaces, which became problematic as a large rule set was generated quickly.
Finally, the chosen model was a piecewise Neural Network algorithm. This was the best as it took all the strengths of the previous three models and dealt with their drawbacks. The piecewise linear equations support the development of a mathematical model, like the statistical regression. It is applicable to nonlinear problem domains like the neural network approach. Finally, due to the piecewise equations in the hidden layers, there is explicit knowledge and formulas unlike the adaptive neuro fuzzy inference system, where it was implicit and categorised.
The results from the study identified the most significant parameters that had the biggest influence in the process’s efficiency and plant performance.
These were found to be steam flow rate through the reboiler, reboiler pressure, and the CO2 concentration in the flue gas. Though these factors are scientific jargon, the methodology bears testament to the contribution that statistical analysis has on the development of more efficient systems.
Whilst we can learn from the case study and innovate new machine learning models to optimise the carbon capture process, there are nonetheless several limitations:
- There is limited data from carbon capture plants. Furthermore, carbon capture plants must be operational in order to build up a deposit of data. This contradicts the primary purpose of switching to a data driven approach; cutting out the emissions associated with a plant’s iterative procedures.
- Even if we did optimise this process, the most cost efficient and secure form of CC may yet underperform in comparison to other emissions reduction techniques such as offsets, green hydrogen, and energy storage.
- Excuse to pollute. Fossil fuel and other emitting companies may use carbon capture as an excuse to pollute, conflicting its primary objective to remove the amount of carbon in the air.
Carbon capture has a long way to go. Currently, the costs disincentivise companies from innovating in the space and as such, the environment has paid for its failed attempts. A data driven approach may prove to be an efficient way to optimise the process, with little cost to the environment.
Actuaries have the skillset and expertise to contribute to this bourgeoning area and have the opportunity to collaborate with scientists to make meaningful change for the planet.
References: [1] https://www.theguardian.com/environment/2021/jul/20/a-shocking-failure-chevron-criticised-for-missing-carbon-capture-target-at-wa-gas-project |
CPD: Actuaries Institute Members can claim two CPD points for every hour of reading articles on Actuaries Digital.