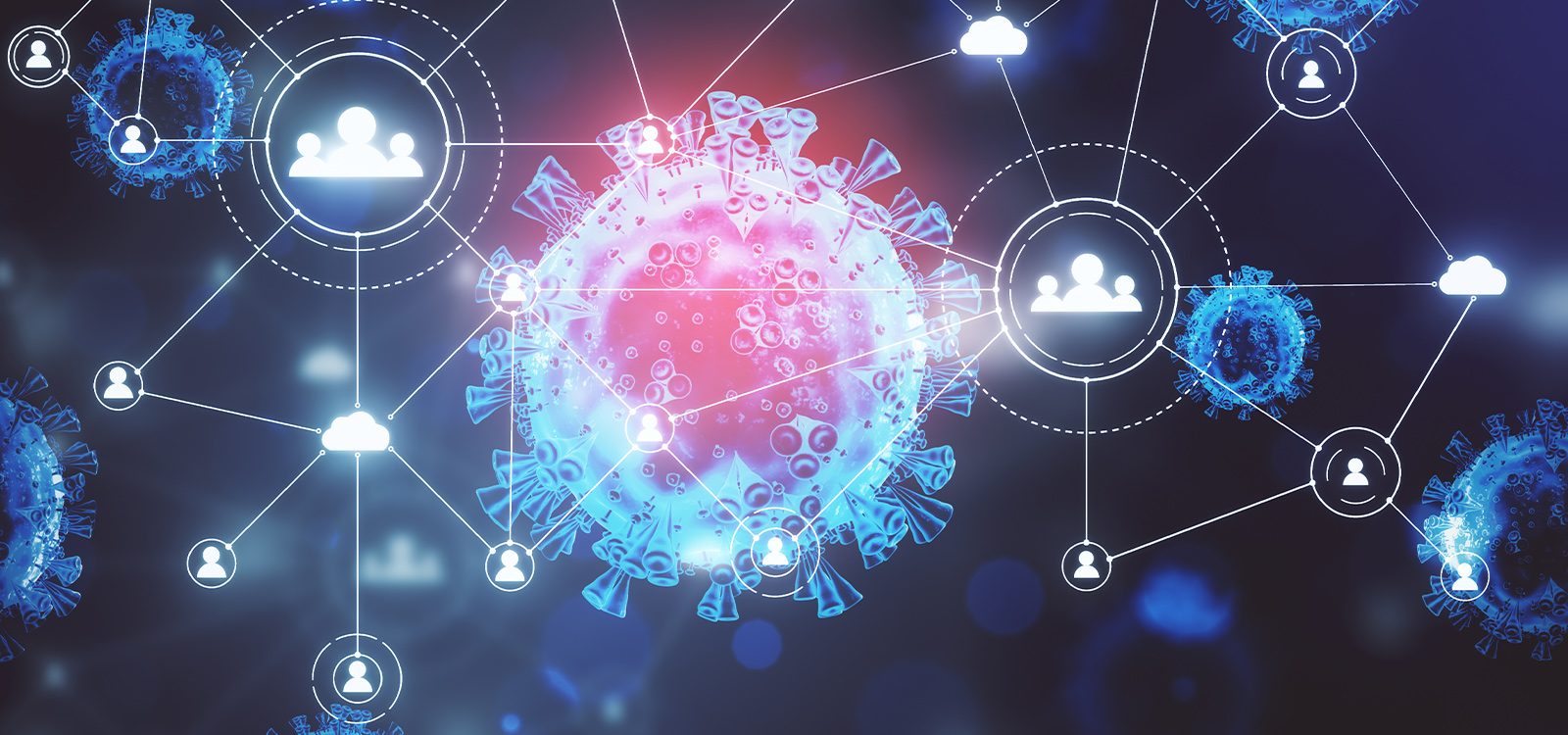
The pandemic – a big win for primary data collection and dashboarding, a loss for AI
In this edition of Normal Deviance, Hugh Miller looks at some of the literature around the use of Artificial Intelligence (AI) during the COVID-19 pandemic and reflects on some of the challenges in creating useful AI tools.
The pandemic has brought many issues into sharp focus. One is the public benefit of good data collection and dissemination – what would be termed ‘business intelligence’ in the corporate world. Worldwide resources such as the Johns Hopkins Coronavirus Resource Centre and the Our World in Data coronavirus hub have allowed people to explore up-to-date information and understand how the pandemic is evolving through various peaks and troughs. Most national governments have similarly invested in data collection and reporting. In Australia, the Commonwealth and State governments publish large amounts of detailed information, often daily. This facilitates the research of others too; for instance, much of the more advanced epidemiological modelling relies on this data as a starting point.
Similarly, the value of good epidemiological modelling has been proven. In Australian organisations such as the Doherty Institute and Burnet Institute have provided advice to government that has directly fed into decisions on the nature and durations of restrictions used to manage the pandemic.
With these successes, it is natural to ask if the high-tech frontier of data science, AI and machine learning, have played similarly useful roles during the pandemic. Unfortunately, the results are not so flattering.
One area of research has been the use of predictive modelling to better identify and triage patients with COVID-19. A report by Wynants et al. (2020) in the British Medical Journal reviewed over 200 of these prediction models. Overall, it found that:
- All models were rated with a high or unclear risk of bias, due to non-representative samples of control patients, sample selectiveness, overfitting and unclear reporting.
- Only 5% of models were externally validated via a calibration plot (to indicate how the model was likely to perform in the wider world).
- Just two models were identified as promising models, worthy for further research.
Therefore, the use of such models as decision support tools is highly problematic.
Another area of research has been the automatic diagnosis of COVID from scan data (mainly chest x-rays and chest CT scans). A review by Roberts et al. in Nature Machine Learning, who found and reviewed 62 such models. They were even more damning – finding that none of the models were suitable for clinical use due to methodological flaws and biases. Again, the risk of bias was generally high, being based on small (and poorly balanced) datasets, and relatively low rates of external validation. More worryingly, many papers did a poor job at attempting to validate the models, and in one case someone accidently used a subset of their training data as the test! In many studies the proposed performance of a tool was judged optimistic, rather than realistic.
What should we conclude from these systematic reviews – do they mean a retreat from AI in medical science? Most experts say no, since the opportunities are profound. However, there will need to be significant scrutiny of AI work to earn the trust of practitioners and patients, particularly following the lack of traction seen in the pandemic and the struggles of other healthcare investments such as IBM Watson. Lots of solutions and improvements have been mooted – much of it relates to better data and sharing, more systematic collaboration with clinicians, more work validating and comparing to other models. Much of this relies on researchers themselves to strive for a higher level of quality so that a publishable result can get closer to a useful one.
And it is important to recognise there have been some other bright spots for AI and big data during the pandemic too. For instance, the Moderna vaccine used AI for mRNA sequence design in vaccine development. Greece used an AI screening system for people entering the country to flag those at relatively low or high risk of having COVID-19, making better use of limited testing resources. And mobility data from tech companies has proven a valuable tool drawn from big data, allowing policymakers an up-to-date forecast of transportation around cities.
With the success of traditional business intelligence, dashboarding and traditional ‘hard science’, it is fair to say the current pandemic is the first global pandemic truly managed by the numbers. But we’re still a fair way away from being able to rely on AI tools to ride to the rescue.
CPD: Actuaries Institute Members can claim two CPD points for every hour of reading articles on Actuaries Digital.