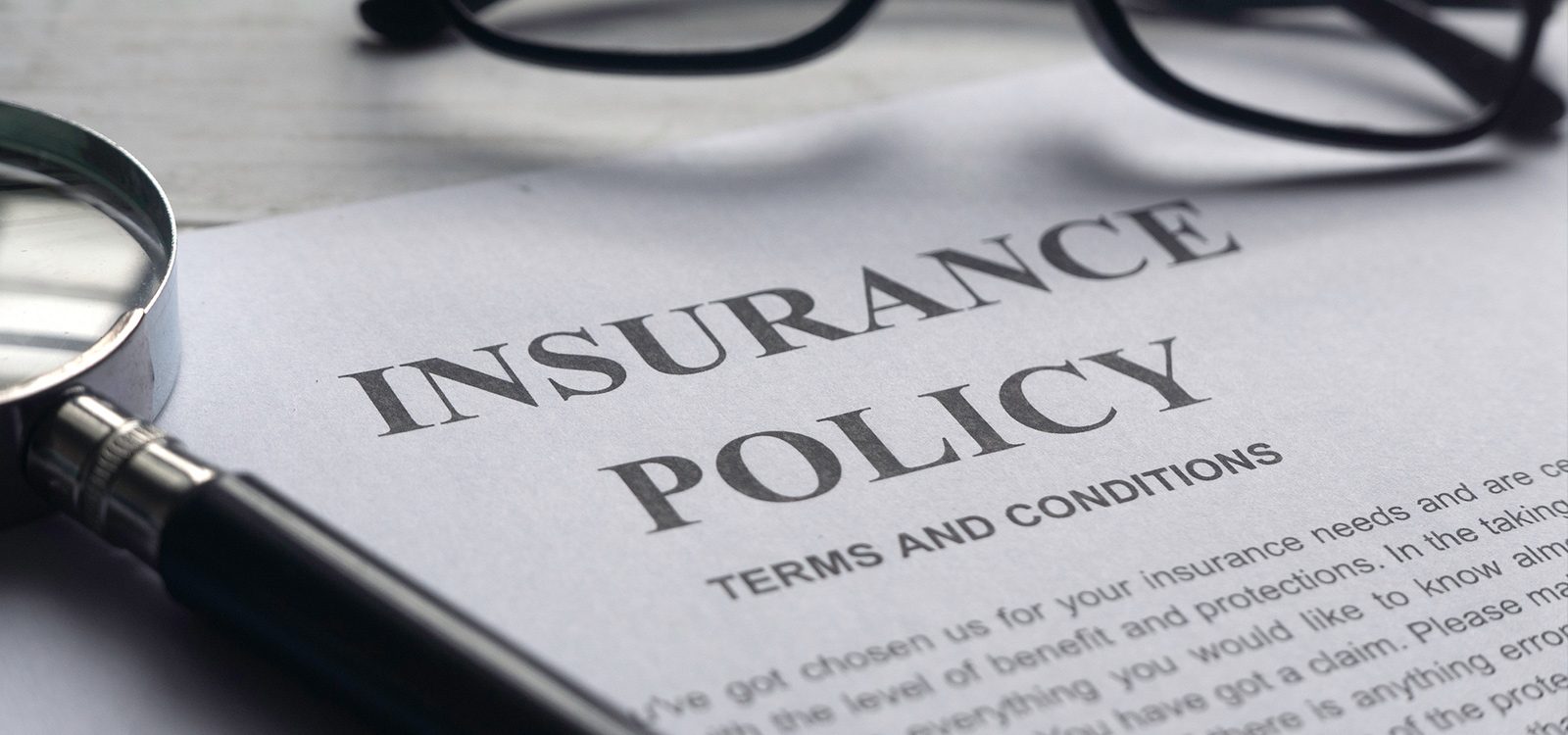
So, you think you can underwrite?
Alex Pui and Maura Feddersen explore the key cognitive biases and other drivers of judgement and decision-making in underwriting, and suggest methods to help underwriters overcome these factors.
From doctors to traders, behavioural economists have researched in detail what helps professionals achieve greater accuracy in their judgement and decision making. Yet, underwriting has not received the same attention. This is surprising, as underwriters are required to make decisions in the face of volatile, uncertain, complex and ambiguous (VUCA) environments, where cognitive biases tend to take effect. In particular, underwriters operating across volatile lines of business, such as large commercial risk sectors are exposed to low frequency and high severity natural catastrophe impacts. They also face data scarcity, adding to the challenge of dealing adeptly with VUCA contexts to arrive at an optimal decision1.
Identifying and measuring the degree of noise and bias in underwriting
To detect and attribute bias as accurately as possible, we set out to do the following:
- A survey that mimicked real-life underwriting cases (such that respondents were not just answering generic questions about ‘day-to-day’ judgement and decision-making, but also answering underwriting specific questions).
- Scoring of bias through reconciling results from ‘test survey’ conditions, underwriter performance metrics and peer benchmarking.
- Text sentiment analysis, using the Twitter ‘BERTweet’ model trained on tweets, to detect patterns in the sentiment of underwriter thoughts relative to costing parameters and performance metrics
What are the key biases in underwriting?
Based on our collective experience, and the aforementioned exercise, the following characteristics of judgement pose the most concern:
Optimism – “I’m sure the client’s flood defences are operational by now“
- Particularly for natural catastrophe/tail-driven lines, underwriters tend to underestimate the likelihood of extreme events. They are also more prone to give more credit than actuarially fair if there are suggestions that retrofitting/protection measures have been installed following a large loss event – even though the robustness of these measures have not been fully tested. Unsurprisingly, optimism bias has been linked to poorer underwriting performance.
Poor calibration – “I don’t know how accurate my costing is, but I’m probably on the conservative side“
- Studies2 have found that underwriters are often not aware of their own costing accuracy – that is, whether they are overly optimistic or conservative in their costing relative to their own historical track record. This issue cuts both ways; underwriters that are optimistic may pick up poorly performing deals, while pessimistic underwriters will leave money on the table. Crucially, without feedback of their costing accuracy, underwriters miss an opportunity to correct their attitudes towards risk.
Noise – “I’m sure my colleague sees this case as I do”
- When any two underwriters review the same case, they may expect their average colleague to diverge by 27% in their costing, however, the actual divergence is as much as three times as large at 79%. Similar to consequences of poor calibration, large unwanted variations in judgement (‘noise’) are costly for re/insurers; overly conservative costing may lead to lost good deals, while costing that is too low, may lead to a profitability problem.
Framing and confirmation bias –“Thankfully, there’s a model to rely on”
- An important step in the underwriting process lies in referrals. A common scenario involves a model output serving as a starting point, with an underwriter adjustment of that output and then a referral following, particularly if larger capacities are to be deployed. A referral underwriter appraising a case as presented is often susceptible to framing bias, which can hide an objective and holistic view. For example, if loss history is furnished – disproportionate importance could be attached to certain characteristics highlighted in the supporting evidence, unwittingly downplaying other key risk factors. Furthermore, the referral underwriter is often afflicted by confirmation bias, where they consider information that confirms their pre-held beliefs about a case.
Boosting underwriting through timely prompts and closing the feedback loop
The crux of any behavioural intervention starts with an acceptance that we are all prone to cognitive biases and how these might undermine our judgement and decision making.
In response, curiosity and scepticism are helpful outlooks for underwriters to adopt and we encourage underwriters to ask questions about the information they work with as well as their confidence in their judgement at key moments in the underwriting process.
For example, if an underwriter’s assessment falls outside of the range they originally anticipated, this ought to trigger self-reflection and further scrutiny. Considering the range of possibilities rather than a single fixed outcome also reminds us of the degree of uncertainty and its drivers. A wide range might prompt us to seek more information and may alert others to take special care during the review. Furthermore, we can remind ourselves that models are not perfect – attaching a level of confidence associated with particular model outputs helps us and others remember how much reliance to place on them.
Consistent with studies3 that show outperformance associated with diversity of thought, explicitly seeking opposing evidence can help us address overconfidence and confirmation bias, while only considering one source of information (e.g. exclusively client or broker information) could be a red flag. At the point where underwriters are forming a view, salient questions can be asked: did you consider a variety of sources with different perspectives? What information is missing, and how did you deal with this? If we are proven wrong in χ years (known as ‘post-mortem’), why might it be so?
Self-awareness of whether an underwriter’s judgements tend to be overly optimistic or overly conservative can encourage self-corrective behaviours. For example, an underwriter making a ‘borderline’ decision, leaning towards writing something with fairly thin deal economics – but who knows about having demonstrated optimistic tendencies in the past, would then rightfully hold back.
Concluding, behavioural economics offers surprising insights that can boost underwriting performance. A natural starting point may involve conducting an assessment of the degree of noise and bias in underwriting, with surveys corroborated by historical performance metrics. Ancillary benefits of this approach include generating buy-in and increased underwriter engagement as it can be an eye-opening experience. Training, tools for decision-making and opportunities to receive feedback can help reduce bias and hence lead to better underwriting outcomes. This is worthwhile, particularly in high uncertainty/non-homogenous regimes, where expert judgement will continue to outperform a purely algorithmic approach but can still benefit from improved consistency in the decision making process.
References
|
CPD: Actuaries Institute Members can claim two CPD points for every hour of reading articles on Actuaries Digital.