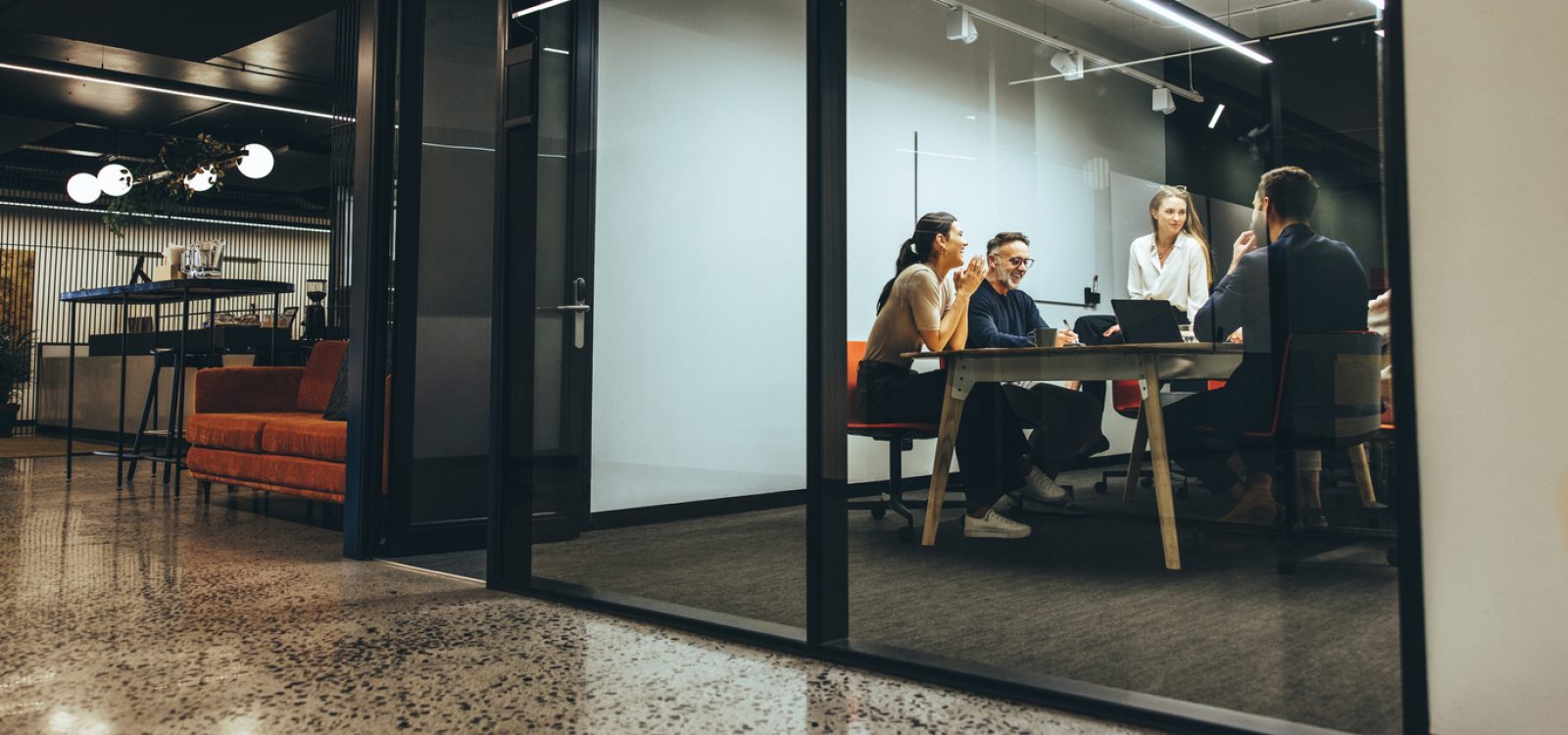
How to spot an effective data science team
I once (quite a few years ago now) had a client who decided to rename his business unit the ‘Analytics Centre for Excellence.’ On one hand, this seemed like a good way to recognise the growing importance analytics to the business unit. On the other – it seemed a large overreach given it was a team with one data analyst with limited coding ability!
Suffice to say that this particular team did not have as large an impact as their name suggested – the main effect seemed to be generating annoyance from other teams that they hadn’t renamed their operations first.
Underneath the anecdote thought is a deeper question I was asked recently, how can you tell if your data science team is a high performing unit?
From the outside, it can be hard to tell and direct comparisons are difficult. I didn’t give a great answer at the time, but this list is the product of a bit more reflection.
Getting the basics right
While the basic functions of data science teams will vary by organisation, the most obvious thing to check is whether core responsibilities are being met with a minimum of fuss. This means that data acquisition, data quality checking, analysis and implementation are all working well (and ideally are becoming more automated over time). A warning sign here can be promises to overhaul processes from scratch – such attempts can end in pain if institutional knowledge baked into existing processes is ignored. By contrast, a team that understands current processes well and is looking to incrementally improve is more likely to be building from a position of strength.
Working with the business
Large organisations carry a lot of interdependencies and complexities. This means that data science solutions need to be compatible. A new whiz-bang model that cannot interoperate with other parts of the business may end up being a significant downgrade, rather than an improvement. Similarly a data science team that hoards data, rather than making relevant data available to other parts of the business may be a red flag.
A natural curiosity in new tools
The data science space, like many others, has evolved rapidly over the past 20 years, and is likely to continue to do so. This includes database design, programming languages, learning algorithms and implementation technologies. While some new fads will pass and will not be worth the investment, many newer approaches contain real value. Having people experimenting with new technologies to sift the shiny from the truly useful is the best way to future-proof a business unit. This includes an interest in both academic developments and commercial innovation.
An appreciation for governance
While data governance can sometimes be the enemy of agility, you do not have to look hard in the news to see the potential pitfalls of lax data practices. Knowing what data you have, where it is stored and who is accessing it is unambiguously core to data science work.
There are many other points that others will advocate – including certain styles of management, team composition and specific tools. But if you’ve got a team that is delivering on the basics, working well across the business, incrementally expanding their capability and managing data safely, then you’re probably in a position of strength.
CPD: Actuaries Institute Members can claim two CPD points for every hour of reading articles on Actuaries Digital.