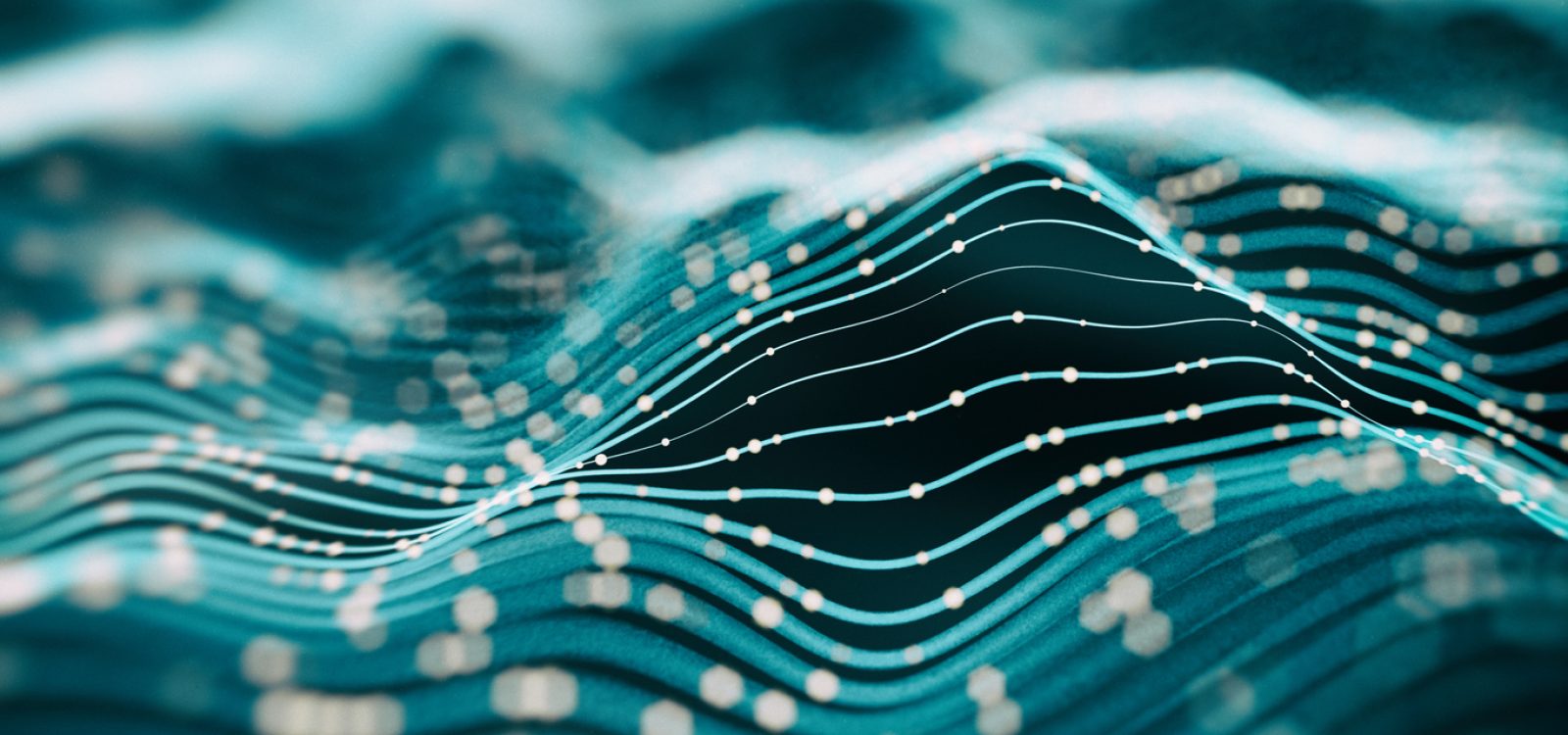
Demystifying reinsurance optimisation
Reinsurance optimisation is an integral part of managing a modern insurance business.
Too much or inappropriate reinsurance can reduce potential profits by passing on profits to reinsurers. Inadequate or ineffective reinsurance can expose an insurer to excessive risk leading to ruin. Therefore, reinsurance optimisation aims to find cost-effective optimal reinsurance protection to meet an insurer’s risk, capital and strategic business requirements. Optimisation is vital in a hard market like the one we are experiencing now.
In a mature market like Australia, it is common practice for insurers, particularly large insurers, to use economic capital models that go beyond capital and reinsurance optimisation. Economic capital models are resource-intensive, time-consuming and costly to implement. Small to medium size insurers need more resources and budgets to implement them. Therefore, reinsurance brokers and other third-party advisors can play an important role in helping insurers optimise reinsurance coverage.
In this article, the focus is on Dynamic Financial Analysis (DFA) in the context of General reinsurance treaty structuring and how DFA can help insurers to optimise reinsurance coverage.
Dynamic Financial Analysis (DFA) – What is it?
Dynamic Financial Analysis is not a new concept in the insurance industry. In fact, this method is prevalent in well-developed markets such as Australia, particularly with internal capital modelling. DFA is a probabilistic simulation approach that looks at an insurer’s risks holistically by analysing and modelling the main financial factors affecting its profit and loss statement and balance sheet. Key financial factors include premiums, losses, acquisition cost and management expenses, reinsurance cost, recoveries and reinsurance commissions.
The DFA process can be carried out to quantify the economic value of different reinsurance structures. The process involves setting up assumptions on key factors based on an insurer’s own historical experience and market-based assumptions where historical experience is sparse. Then these key factors are modelled with thousands of simulations against different reinsurance structures. Modelling produces not just the average but extreme results that can be considered in deciding an optimal and cost-effective coverage.
Optimising reinsurance structures with DFA
DFA involves several steps, including data preparation, assumption setting, modelling, collating and interpretation of results. The following diagram shows how the process is carried out with the above.
Data preparation
Data is one key element attributable to the success of the DFA process. Just as the adage garbage in, garbage out, without quality data, it is difficult to reap benefits from the DFA process. The following list provides basic data requirements for reinsurance optimisation with the DFA process.
- Risk and loss profiles of classes of business covered by the reinsurance structure under consideration. Ideally, five years of historical profiles are required.
- Natural catastrophe exposures of in-force policies applicable to the treaty by different types of peril.
- Historical individual non-catastrophe losses for five to ten historical years. From Ground Up (FGU) losses above a selected threshold with breakdown by ceded percentages into different applicable treaties and facultative arrangements. For long-tail classes, loss development of individual large losses.
- Historical catastrophe event losses of at least ten historical years with breakdown by ceded percentages into different applicable treaties and facultative reinsurance arrangements.
- Reinsurance treaty statistics for at least 5 historical years.
- Estimated Premium Income (EPI) for proportional treaties and Estimated Gross Net Premium Income (EGNPI) for non-proportional treaties. Historical actual/revised premium income/gross net premium income for 5 to 10 historical years.
- Historical treaty structures (e.g. maximum retention, one-line retention of proportional treaties and deductible and limits of non-proportional treaties)
- Original acquisition cost and management expenses by classes of business.
Assumption Setting and Modelling
The following points describe how assumptions are usually set on these key factors.
- Premiums are projected for the next treaty year based on EPIs and EGNPIs.
- Acquisition cost and management expenses can be derived from the latest financial statements or based on information provided by the insurer. Usually, reinsurance commission terms come from expiring treaty terms.
- Generally, losses are segregated into more frequent small losses, infrequent and high severity non-catastrophe large losses, and catastrophe losses for their distinctive risk characteristics. Statistical distributions are fitted to large losses and a best fit is selected. Where historical losses are sparse, an exposure-based method can be used with risk profiles and exposure curves. Typically, large loss frequency is modelled with a Poisson distribution, and small losses are modelled with a Log-normal distribution.
- Typically, catastrophe losses are modelled with commercial catastrophe modelling tools. Modelled losses produced in the form of Event Loss Tables (ELT) or Year Loss Tables (YLT) become inputs to the DFA model. When catastrophe models are not available for a particular peril, we may rely on historical event losses. In some cases, historical losses and catastrophe modelling results are blended to model catastrophe losses.
Model Calibration and Convergence of Results
Just as performed with Internal capital models to ensure that a DFA model produces reasonable outputs, it is crucial to calibrate the DFA model through assumption setting and validating model results. It is essential to carry out a large number of simulations to ensure the convergence of results and to allow for a wide range of possible scenarios with simulations. This is especially applicable to models with a large number of catastrophe events to ensure that each event has a possibility of being picked up for simulation.
Technical Pricing of Reinsurance Structures
Technical pricing of reinsurance structures is an important part of optimisation. It is recommended to use technical pricing across all structures being tested, as the inconsistent application of subjective elements to pricing can distort results leading to the selection of a less optimal structure. Technical pricing can also help to provide guidance on the market pricing of reinsurance structures under consideration. An insurer with technical pricing of reinsurance structures will be in a better position to negotiate market pricing with reinsurers and brokers and cross-validate market pricing, particularly in a hard market like the one we are experiencing now.
Model Results
One example has been used throughout this article to explain modelling outcomes. In the example, an expiring non-proportional reinsurance treaty structure covering risk and catastrophe event losses of Fire, Engineering, Motor and Marine classes of business (i.e. a Whole Account Excess of Loss (XoL) treaty) has been tested against three different strategies as given below.
Strategy 1 – is the same as the expiring Whole Account XoL treaty structure, except the total limit is reduced to provide coverage for losses up to 1 in 250 year return period level from the expiring limit of 1 in 1000 year return period level.
Strategy 2 – introduces individual XoL treaty structures for Fire/Engineering, Motor and Marine classes instead of Whole Account XoL.
Strategy 3 – assumes that there is no Fire proportional treaty, which is inured to the benefit of the expiring Whole Account XoL treaty, and uses the same structure as Strategy 1 but with an increased total limit to provide coverage for losses up to 1 in 250-year return period level. The limit increased as a result of an increase in retention of losses without the Fire proportional treaty.
Analysing model results is a key aspect to the success of the DFA process. It is paramount that results are communicated to stakeholders, especially to non-technical stakeholders, in a manner they can understand. With DFA model results, the main focus is on net underwriting results in the profit and loss statement.
Charts and graphs are one way to communicate model results. With the example given below, a Risk and Reward chart has been set up to compare modelled results with the expiring Whole Account XoL treaty structure and the three strategies based on two measures, standard deviation and an average of net underwriting results.
A basic aim of reinsurance structuring is to:
- Reduce the standard deviation of net underwriting results represented by risk, i.e. move from right to left on X- axis, which represents the standard deviation of net underwriting results and
- Improve average net underwriting results represented by rewards, i.e. moving from bottom to top on Y- axis, which represents average net underwriting results.
Based on the Risk and Reward chart, three strategies improve net underwriting results compared to the expiring structure. However, the improvement comes with increased risk (i.e. an increase in the standard deviation of net underwriting results). An insurer may opt for Strategy 3 for its superior rewards despite the increase in risk. For risk, other measures such as Value-at-Risk (VaR) or Tail VaR can also be used.
The below chart is only for illustration purposes to show that there exists an efficient frontier (blue line) of net underwriting results similar to that of the Capital Asset Pricing Model (CAPM) corresponding to different reinsurance structures when average net underwriting results are plotted against the standard deviation of net underwriting results[1]. Theoretically optimal net underwriting results lie on the efficient frontier. It is not practical to produce the efficient frontier as it involves modelling many different reinsurance structures, which is time-consuming and computationally intensive. Therefore, it is pragmatic to select several options and carry out the DFA process to save time and effort.
Some insurers may look at more measures than the two mentioned above to compare different reinsurance structures. In this case, a radar chart is a better way to represent results. The following radar chart for the same example shows the comparison of structures with six different measures. In the radar, chart scale-1 represents the worst outcomes, whereas scale-5 represents the best outcomes. The lowest ceded ROE, superior capital savings and the best upside may compel an insurer to consider Strategy 3 over others.
Results can be presented in tabular form to show detailed information. The following table shows the profit and loss statement of classes, of businesses under consideration in the example. Underwriting results before and after applying reinsurance strategies are shown with parts making up underwriting results. Apart from measures presented earlier, additional information such as best-case and worst-case outcomes of underwriting results at different return periods, have been presented in the table. For example, there is a 0.4% probability (i.e. 1 in 250-year return period level) that net underwriting losses will be USD 14.73 million or more with the expiring structure. The downside results before and after applying reinsurance structures show the effectiveness of reinsurance structures in helping to reduce volatility and save capital. For example, without reinsurance protection, the capital requirement at 1 in 250-year return period level would have been USD 127.41 million compared to USD 14.73 million with the expiring structure.
Advantages and disadvantages of DFA process
The DFA process comes with its own advantages and disadvantages. They include some of the following.
Advantages
- Since the DFA process typically uses an insurer’s own experience, modelling outcomes fit well to reflect the insurer’s own risk profile.
- Can assess the impact of a strategy. This can be achieved with the quantification of the impact of reinsurance structural changes on profit and loss statement.
- Provides a wide range of measures to consider in deciding on a reinsurance structure based on an insurer’s own risk appetite, capital and business requirements. Hence provides transparency to decision-making.
- Can be extended to facilitate internal capital model development.
- Technical pricing of reinsurance structures can be used as a reference to help negotiate with brokers/reinsurers for better pricing.
- Can assess the impact of Realistic Disaster Scenario (RDS) events on different reinsurance structures.
- Can assess the adequacy of horizontal coverage of non-proportional structures (e.g. number of reinstatements required)
- Complex features such as correlations and tail dependencies (with copulas) between classes of business can be set up with DFA modelling tools.
Disadvantages
- A more complex and time-consuming process.
- Costly as the modelling process requires special tools to develop and implement.
- A lot more emphasis is put on data quality. Outputs from the model are as good as the input data to the model.
- Requires specialised skills to develop and implement and they can be costly and scarce.
- Prone to model errors, parameter errors and process errors adversely impacting model results.
At present, we are experiencing a hard market with significant reinsurance cost increases. Therefore, reinsurance optimisation has become even more important than in the past in finding cost-effective optimal reinsurance protection. Dynamic Financial Analysis (DFA) process can be used for assessing reinsurance strategies. Despite being data-intensive, time-consuming and costly, the DFA process provides more benefits to insurers in assessing reinsurance structures. Good data quality, relevant skills required for the DFA process and the communication of modelling outcomes in a way stakeholders can understand are keys to reaping full benefits from the DFA process for reinsurance optimisation.
References
- “Optimal Layers for Catastrophe Reinsurance” – by Luyang Fu and C. K. “Stan” Khury, (http://www.variancejournal.org/issues/04-02/191.pdf)
Further Reading
- “Reinsurance Optimisation in the Context of Risk Management and Solvency Regulation” – by Eva Schläpfer de Montmollin, Group Financial and Risk Modelling Group, 6th July 2010.
- “Reinsurance Optimisation, GIPC Reinsurance Working Party” – by Steven Girvan and Will Gardner (https://www.actuaries.asn.au/Library/Events/GIS/2012/3ai_StevenGirvan.pdf)
- “Making use of DFA (Dynamic financial analysis)”, https://actuaries.asn.au/Library/Events/Conventions/2007/7.d_Conv07_Paper_Nicholls_The%20Reality%20of%20DFA.pdf
Special Note: Authors would like to thank Mudit Gupta (FIAA) for reviewing the article and providing invaluable feedback.
CPD: Actuaries Institute Members can claim two CPD points for every hour of reading articles on Actuaries Digital.