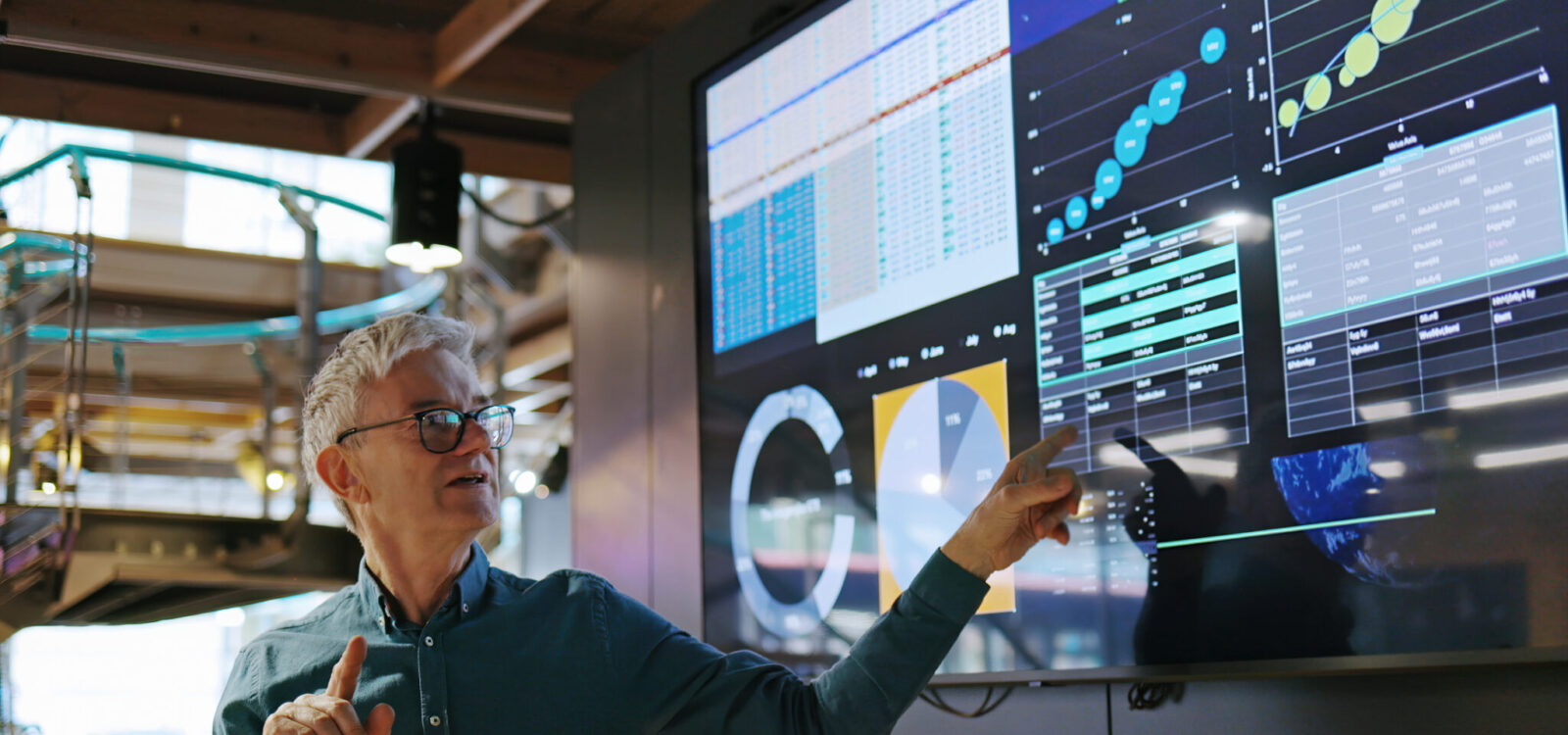
Measuring Things the Right Way
In this Normal Deviance column, Hugh looks at some instances of misalignment between measures and the underlying questions they need to answer.
One of the (thankfully relatively few) times my work has been publicly criticised was our work estimating lifetime welfare trajectories in New Zealand. At its core, the work estimated how long people would stay on benefit and use decreases in that estimate as evidence of improved management of the income support system. The logic was that movements off welfare correspond to increased employment and income and so, reflect economic gains for both individuals and government.
A key criticism was around this use of welfare exits as a proxy for improved outcomes. What if a policy change forced people off benefits even if they did not find a job? People’s wellbeing would suffer, but long-term welfare spend would reduce.
At the time, we were aware the measure could be used poorly and so were careful to consider this risk as we went about modelling changes over time. However, the basic point was right – we could only see a proxy indicator (exit from welfare) for what we really wanted to measure (improved employment and incomes). Happily, this is a piece of work that was able to evolve – good linked data in New Zealand means that we can now track people’s incomes after they exit welfare to reduce potentially invisible poor outcomes.
There is a broader lesson here (aside from the fact that I am clearly very slow to shrug off criticism): very often we deal with data that does not align well with our true intended outcome. Tracking interactions with specialist homelessness services is only a proxy of broader homelessness, since many homeless people do not interact with services. Insurance exposures to extreme events gives only a partial view of the true costs of these events, given significant rates of non-insurance and underinsurance. Customer spending patterns at a particular store give only partial insight into loyalty since spending outside the store is not visible. Online shopping purchases are notoriously hard to properly link advertising and other marketing efforts. Our view of systems is often proxied and incomplete.
For actuarial work, this issue ultimately leads to what datasets are available for an analysis. Early in my actuarial career, I viewed our default position as ‘data-takers’, where we had little control over what information was collected and our task was to make the best of what we knew. In many traditional contexts this was reasonable; the data available was appropriate to the purpose and we were able confirm completeness and consistency.
Over time, I’ve come to appreciate that, in many cases, we have greater agency than just accepting available datasets.
If there are gaps in the data, we can run a survey or other secondary collection. If an adjacent data source provides insight, it can be brought in, and sometimes even joined together to augment the primary datasets. And we can advocate for improvements in data collection and management for future work. Pleasingly, it has been getting quicker and cheaper to collect additional relevant data.
The ability to go beyond acceptance of existing datasets is important to keep in mind. We should be alert to situations where our data does not line up well and seek to understand if we can do better. We should also watch out for the same issue when using other people’s findings.
This brings me, (very) circuitously, to mental health. This is an issue that intersects with many areas of actuarial work; life insurance, health insurance, workers’ compensation and non-traditional areas are all affected by the prevalence of mental health injuries in a customer base. Significant effort goes into identifying people with mental health needs, and how this translates to increases in claim frequency and severity (or influences other outcomes of interest).
However, too often a mental health injury is a flag used to inform predictions and little else.
Case managers might tailor supports to the issues, but the data captured will not track recovery of a mental health injury, instead using other proxies (such as return to work, or cessation of psychological supports) as an indicator of success.
Compare this to a world where some measure (or set of measures) of psychological distress is regularly captured to understand the evolution of a mental injury, plus a customer’s view on recovery and salience. With this, more direct measurements of impact can be tracked to understand how treatments and other supports are helping a person., and typical patterns in distress can be understood (e.g., if injuries naturally resolve over time for some customer segments) and linked to other outcomes. It would also enable better comparison and consistency in treatment.
This type of work does sometimes happen – this article is obviously not the first call for better data on mental health injuries. But we can do better. Treat this as a call for us to continue to push for improved data, particularly when our current proxies do not tell the full story. You might even save yourself a haranguing in the media.
CPD: Actuaries Institute Members can claim two CPD points for every hour of reading articles on Actuaries Digital.