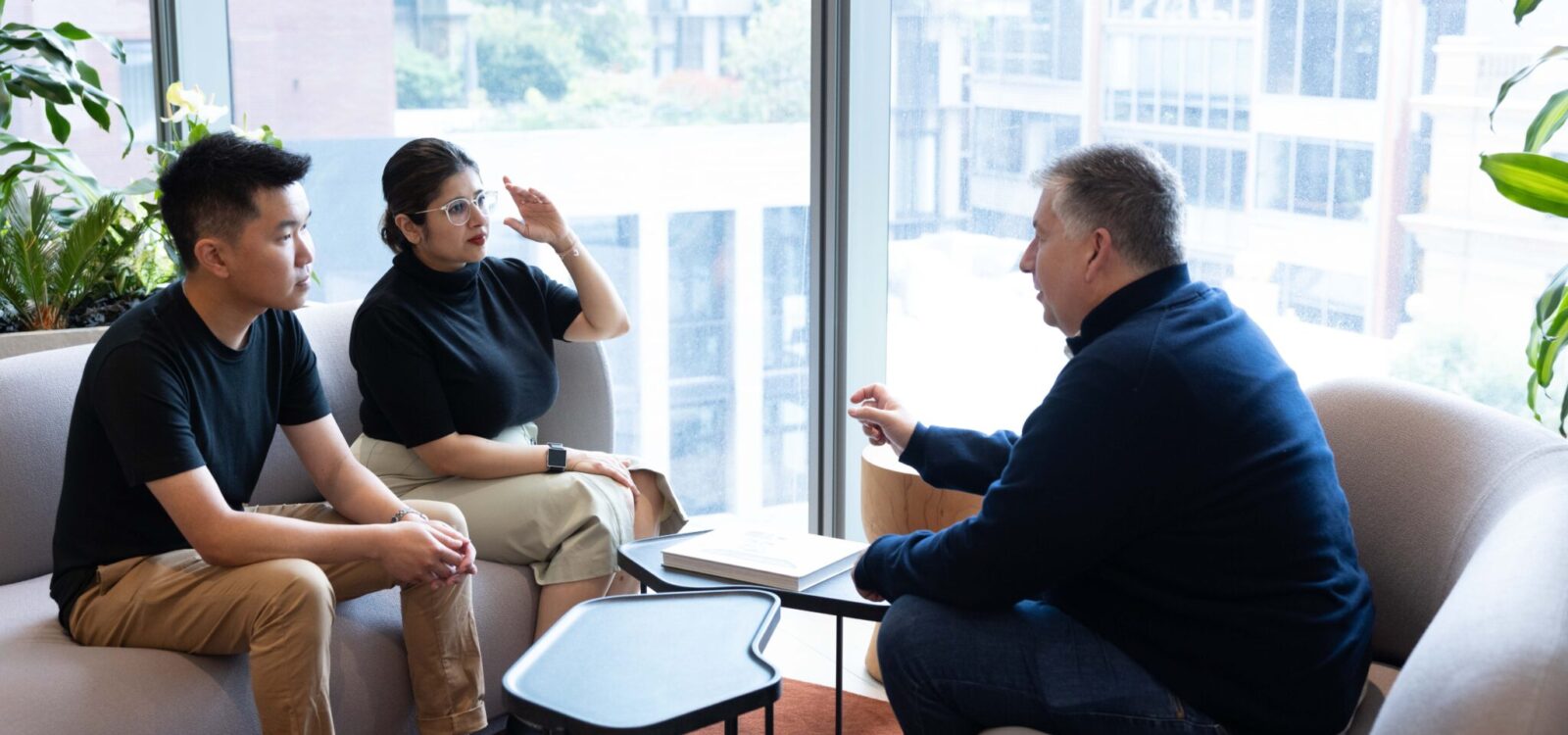
Nature offers insight into bank operational risks
What has biological evolution got to do with operational risk in Australian banks? As PhD students at Sydney Business School are discovering, quite a lot. Actuarial Professor John Evans reports.
Both biological evolution and financial institutions operate in what is known as complex adaptive systems. The essential characteristics of a complex adaptive system are that:
- it consists of interconnected components;
- the interconnections lead to behaviour that cannot be observed in the constituent parts;
- the system adapts to inputs and evolves; and
- the system contains uncertainty.
There are significant parallels between the complex adaptive systems financial institutions operate in and those in which biological evolution occurs[1]. Whereas in biological evolution natural selection removes poor characteristics, in operational risk, the parallel is management intervention to remove unwanted risk events. More examples of this can be found in Josh Corrigan and Neil Allan’s paper on successful adaptation of evolutionary analysis to financial institution risk events, presented at the Actuaries Summit in 2013[2].
A PhD program to further the research into the adaptation of the techniques used to understand biological evolution to capital markets has commenced at the Sydney Business School, in conjunction with Neil Allan of the Systems Centre at Bristol University in the UK.
Three PhD candidates are researching the application of evolutionary analysis to operational risk, credit risk and market risk. They will present their findings in relation to operational risks in Australian banks to Actuaries Institute Insights Sessions in Sydney, Melbourne and Canberra over the next month.
The traditional quantitative models of risks in financial institutions are aimed at defining the range of losses arising from likely risk events over reasonable time periods. The problem with using this type of analysis here is that financial institutions operate in ever changing environments. This makes statistical analysis difficult as the results of the analysis are time dependent. The statistical analysis also offers no insight into why the risk event is occurring and is therefore of little value to those trying to manage it.
The technique being used in the analysis of the Australian banks’ operational risk events is as follows.
Firstly, we identified from the descriptions of the risk events provided by the banks in the Riskbusiness Oprisk Intelliset, a global database of operational risk events, the main characteristics of the risk events. We then used a particular analysis known as phylogenetic analysis to sort the risk events by their characteristics in a hierarchical manner such that the families of risk events described by common characteristics were grouped together. This process produces a tree with risk events on the right and then groupings by the various common characteristics proceeding to the left until there is a single common characteristic for a group of risk events, with the number of these most common characteristics minimised. These minimised common characteristics are referred to as “Tier 1” characteristics.
As the trees derived from the analysis trace the common characteristics of all captured events, the trees are quite large. The tree below illustrates the situation where the most common characteristic was “poor controls”:
In carrying out the analysis, we considered stability over time, particularly of the Tier 1 characteristics was important, as this would mean the banks could then rely upon these characteristics as being features of the risk events, and concentrate on managing these features to help reduce losses to an acceptable level in the future.
The following table shows for the analysis of the Australian banks’ operational risk events the most common Tier 1 characteristics, where these are the most common characteristics that are related to the risk events such that there are the least Tier 1 characteristics.
Tier 1 characteristics of phylogenetic trees by year
|
Whole tree |
Trees separated by year |
||
|
2010-2014 |
2010 |
2011-2012 |
2013-2014 |
Characteristics |
Poor controls |
Poor controls |
Poor controls |
Internal fraud, External fraud, Multiple people |
Legal issue |
Legal issue |
Legal issue |
Crime, ATM |
|
Single person |
Single person |
Single person |
Poor controls, Complex transaction, Manual process |
|
Crime |
Crime |
Crime |
Poor controls |
|
External fraud |
External fraud |
Multiple people |
Poor controls, External fraud |
Whilst there are some changes in these Tier 1 characteristics over the time periods analysed, there is significant consistency, which means the banks can concentrate on these issues to reduce losses if they want to. Just to illustrate the importance of these Tier 1 characteristics in creating operational risk event losses, the following table shows the percentages of total losses from each characteristic:
Ranking of Risk Event Losses by Characteristics
Characteristic | Percentage of Total Losses
Poor controls – 60.1%
Internal fraud – 27.7%
Legal issues – 22.8%
Regulatory issues – 16.6%
Bank cross-selling – 11.4%
External fraud – 9.7%
Overcharging – 8.1%
Crime – 7.0%
Money laundering – 1.0%
Misleading information – 0.4%
Employment issues – 0.1%
Computer hacking – 0.0%
Human error – 0.0%
There is little doubt that poor controls are a major source of losses for Australian banks. This new type of analysis has promise in being able to assist management to identify the main characteristics of their operational risks which has not been possible previously, and the analysis of the operational risks for Australian banks is showing stability in the Tier 1 characteristics, which means these are likely to be the characteristics going forward and which can then be targeted for control to reduce risk losses.
Preliminary results for US and European banks are showing similar but not identical Tier 1 characteristics for operational risk events. One intriguing result for non-Australian banks is that just being a “big bank” is showing up as an important characteristic of operational risk losses. It was not possible to use this characteristic in the Australian bank analysis as the database consist entirely of operational risk events for the major banks.
[1] Allan N, Cantle N, Godfrey P, et al. (2012) A review of the use of complex systems applied to risk appetite and emerging risks in ERM practice. British Actuarial Journal: 1-72.
[2] Allan N and Corrigan J (2013) Emerging Risk Assessment-Latest Practice & Innovation. Actuaries Summit. Sydney
CPD: Actuaries Institute Members can claim two CPD points for every hour of reading articles on Actuaries Digital.