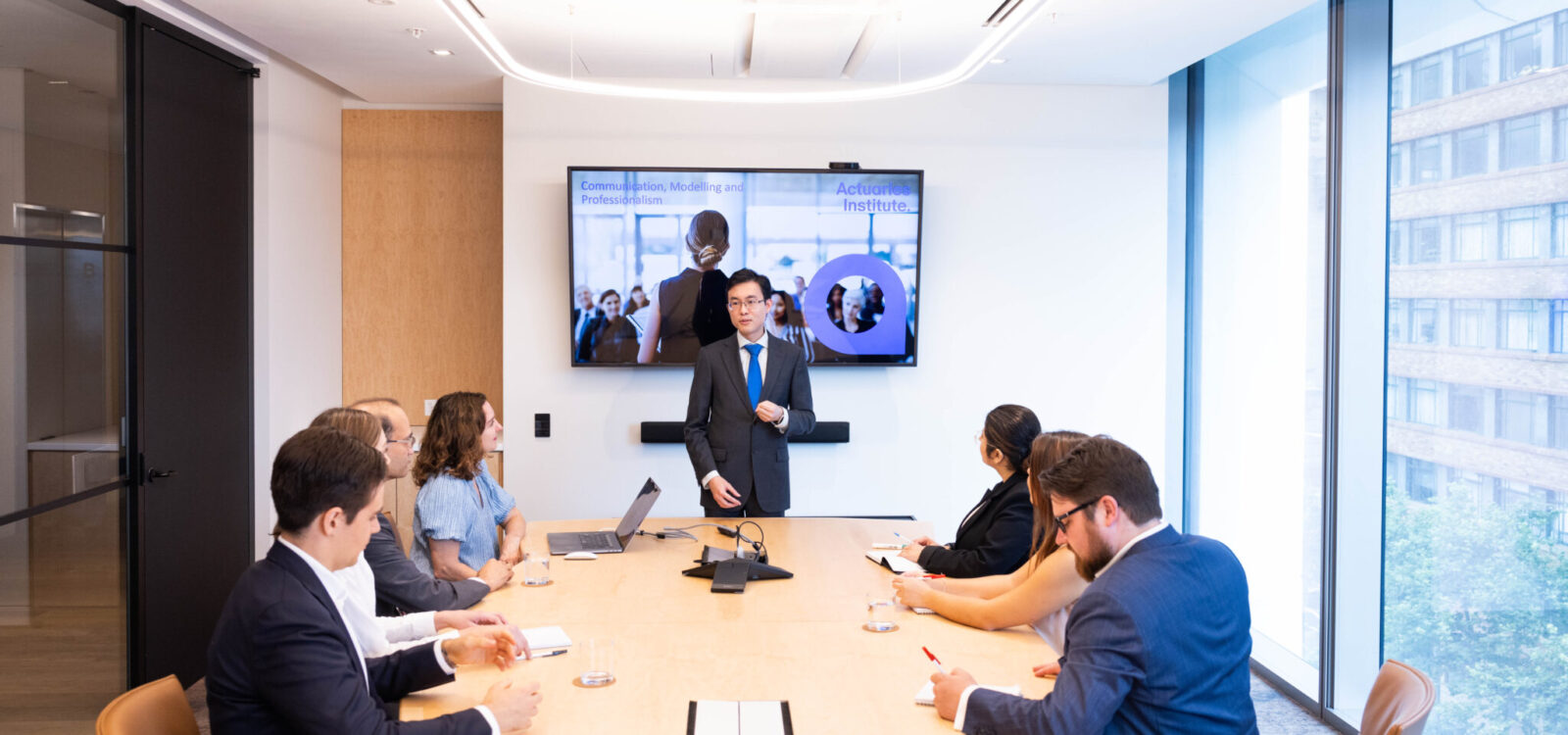
Analytics-assisted triage of workers compensation claims
Director of Data Science Inna Kolyshkina and Scheme Actuary at ReturnToWorkSA Ivan Lebedev combine forces to explain a project undertaken to explore the usefulness of advanced data analytics capability for ReturnToWorkSA.
In 2014, ReturnToWorkSA undertook a project to explore the potential usefulness of advanced data analytics capability to its business.
The aim was to predict the likelihood of claims staying on income support for one year or more from the date of lodgement (hereafter, this event will be referred to as “becoming long-term”) using the information available at thirteen weeks from lodgement.
A further requirement was that the prediction model should be easily interpretable by the business.
On average, by 13 weeks after claim lodgement, more than 80% of claimants will have returned to work. The remaining ones must have had certain barriers that prevented them from making a recovery. These barriers are commonly related to the severity of the underlying medical condition, psycho-social factors such as the relationship with employer/job, worker’s general resilience etc.
At 13 weeks post-lodgement claims establish a history that includes medical diagnosis and treatment, interactions with GP/specialists, entitlement payments, etc. While each element of this data may not be particularly predictive, the business case set out to check whether advanced data analytics would allow one to identify the patterns and combinations that reliably predict high or low probability of a claim becoming long-term.
Challenges
The event of a claim becoming long-term is influenced by many factors. Strong variability of claim duration for a given injury type and age is illustrated in Figure 1.
Figure 1: Two-way analysis of claim duration versus age and nature of injury. The blue curve shows generalised additive model (GAM) fitted in the data and the dark grey region around it shows the corresponding confidence interval band. A high degree of variability for injured workers of the same age and injury type is clearly visible.
The features that significantly complicate the modelling of claim outcomes are data sparseness, multicollinearity and the fact that the majority of the potentially important predictors (such as TOOCS codes for nature of injury, body location, etc) have large number of categories.
Facing the challenges
TOOCS system has a wide gap between the highest level (nature of injury group) and the lowest level (individual nature of injury). As a result, some of the high-level categories are too broad to be useful, while some of the low-level categories have too little support (number of claims in the dataset). To address this situation, low-level categories with large support were raised up in the hierarchy, high-level categories with small support were lowered down and low-level categories with small support were amalgamated with similar ones.
Since nature of injury and body location were expected to be amongst the most important predictors of claim duration, an important step was to combine them into a single variable in order to concentrate only on combinations that occurred in practice.
Finally, we applied correlation analysis to identify the clusters of variables that were highly correlated to each other; the variables that were found to contain redundant information could be removed from the analysis without sacrificing the accuracy or validity of prediction.
Early disappointment
To efficiently evaluate what accuracy could be achieved with the chosen predictors, we employed three different data science methods known for extracting maximum predictive value from the data – Random Forests, GBM and LASSO regression.
The results were consistent for all the methods used and showed that only 11-13% of the variability as measured by R-squared- equivalent measures was explained.
The segmentations performed by Conditional Inference Trees, classical Classification and Regression trees and cluster-based approach were consistent in producing only two main claim segments with poor separation between the probability of a claim becoming long term (Figure 2).
Figure 2: Initial segmentation of claims. The separation between high-risk and low-risk segments is low.
This result clearly did not meet the business expectation.
1 Data enrichment
This result indicated that certain unknown factors excluded from the initial model influence the outcome. Using the input from the SMEs and external research in worker compensation claim duration prediction, we then sought to enrich the data with additional information, including:
• claim reporting lag;
• information on the treatment received (for example, type of providers visited, number of visits, provider specialty);
• information on the use of medications and, specifically, on whether a potent opioid was used;
• information on claimants’ prior claim history, including previous claim count, type and nature of injury and any similarity with the current injury
There was a significant increase in the proportion of variability explained by the model.
We identified 36 most significant attributes for classifying claims into high- and low-risk segments. The top 12 predictors are shown in Figure 3.
Figure 3: Top 12 predictors for the risk of a claim becoming long-term. The green line shows the extent of the importance of each predictor on the scale from 0 to 100.
Building the final model
The business required the probability of a claim becoming long-term to be expressed in the form of intelligible business rules. To achieve this, we used Decision Trees in combination with Association Rules analysis.
The final model allows one to allocate a claim to one of 6 segments shown in Figure 4 on the basis of 36 characteristics and their combinations.
Figure 4: Segmentation of claims by the final model
The model shows a good separation between the high-risk segments (1 and 2) and low-risk ones (5 and 6). The ability to reliably identify claims with high risk of becoming long term has a clear business value because it can be used to focus case management activity where it is most needed.
Key learnings
Although one might think that decision tree-based methods could work with raw categorical data and that the binary splitting algorithm would automatically amalgamate small categories into larger groups, in reality, this is not the case. Our experience in this and other projects is that a thorough review, cleansing and regularisation of categorical data is essential for building a good prediction model.
The appreciation of the critical role of expert business knowledge in achieving good outcomes was another key learning. It is by consulting the subject matter experts that we were able to identify that the history of prior claims can be added to the model. This allowed us to significantly improve the prediction accuracy.
The approach that worked very well in this project was to first focus on achieving a satisfactory prediction accuracy and then concentrate on developing the final model that meets specific business requirements. When targeting accuracy, by using the tools that extract the greatest amount of predictive power from the data we could quickly assess the inadequate predictive potential of the initial dataset and direct our efforts to data enrichment.
At the stage of developing the final model we already had a defined set of predictors to work with and could concentrate our efforts on refining the model itself. It should be noted that depending on the business requirements, the final model could have been developed not only in the form of decision rules, but also in any other form (e.g. GLM) required by the business.
CPD: Actuaries Institute Members can claim two CPD points for every hour of reading articles on Actuaries Digital.